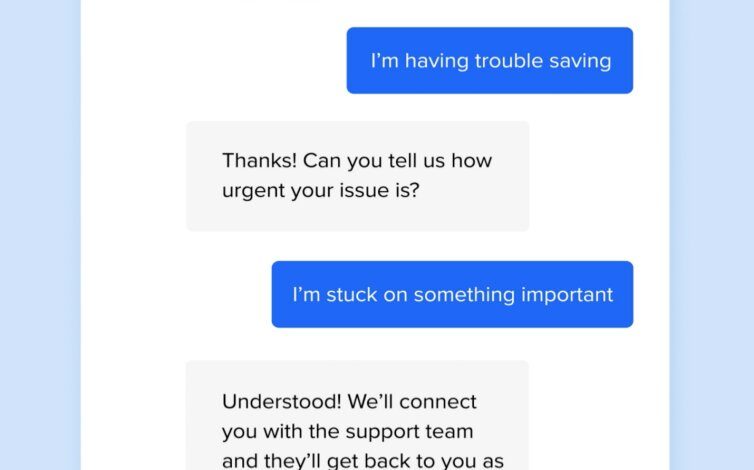
The Best Chatbots Examples A Deep Dive
The best chatbots examples are revolutionizing how we interact with technology. From customer service to entertainment, these AI-powered assistants are rapidly evolving, offering unprecedented convenience and efficiency. This exploration delves into the core components of exceptional chatbots, analyzing their strengths, weaknesses, and the critical role of user experience in their design. We’ll examine the technical aspects driving their capabilities, and even glimpse into the future of chatbot development.
This in-depth look at the best chatbots examples will cover everything from defining what makes a chatbot “best” to examining specific use cases and the underlying technology. We’ll also discuss the crucial role of user experience and interface design, as well as the technical capabilities that enable these amazing tools. Ultimately, the goal is to understand what makes a chatbot truly exceptional.
Defining “Best” Chatbots
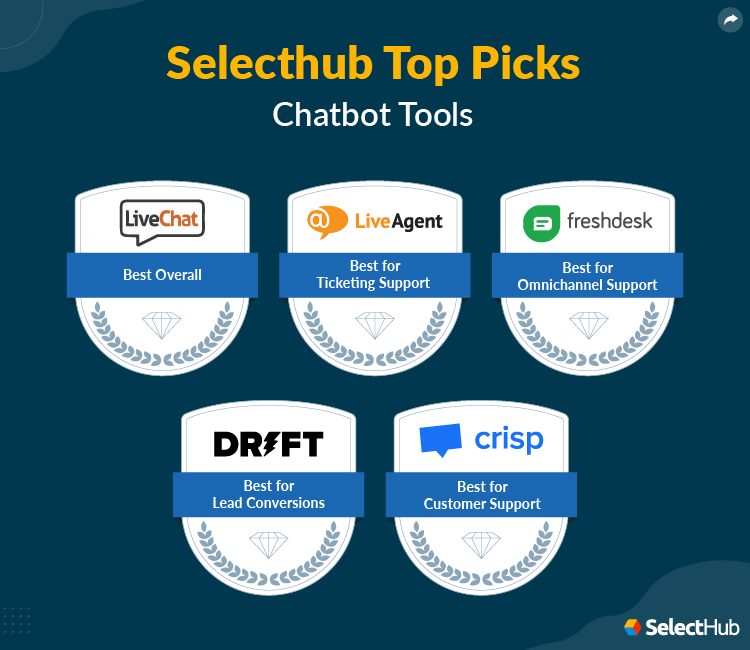
Defining the “best” chatbot is a multifaceted endeavor, moving beyond simplistic comparisons. It requires a comprehensive understanding of user needs, technical capabilities, and the specific context of application. A truly “best” chatbot seamlessly integrates functionality, user experience, and technical prowess to deliver value and meet user expectations.A “best” chatbot isn’t just about sophisticated algorithms; it’s about intelligent interaction and a positive user experience.
This involves not only accurate and relevant responses but also a natural, conversational flow that feels intuitive and helpful. Crucially, the best chatbots adapt and learn, continuously improving their performance based on user interactions and feedback.
Criteria for Evaluating Chatbot Effectiveness
Evaluating chatbot effectiveness necessitates a structured approach, encompassing several key metrics. Accuracy, measured by the precision and relevance of responses, is paramount. Speed of response directly impacts user satisfaction and experience. Natural language processing (NLP) proficiency determines how well the chatbot understands and interprets human language, impacting the quality of interactions. Ultimately, a chatbot’s success hinges on its ability to efficiently and effectively solve user problems.
Benchmarks for comparison should include metrics like user satisfaction scores, task completion rates, and the time taken to resolve issues. These metrics provide a robust framework for objectively assessing chatbot performance.
Different Types of Chatbots and Their Strengths and Weaknesses
Chatbots come in various forms, each with unique strengths and limitations. Understanding these distinctions is crucial for choosing the right chatbot for a specific task. Rule-based chatbots, for example, excel in situations where responses are predefined and predictable. However, their adaptability is limited. Machine learning (ML) chatbots, on the other hand, possess a higher degree of flexibility, learning from interactions to provide more nuanced and personalized responses.
The best choice depends on the specific needs of the application.
Examples of Chatbot Categories
Chatbots find applications in diverse sectors. In customer service, chatbots handle routine inquiries, freeing up human agents for more complex issues. In education, chatbots offer personalized tutoring and support, adapting to individual student needs. Entertainment chatbots can engage users in interactive games and conversations, enhancing user experience. These examples showcase the versatility and potential of chatbots across various domains.
Comparison of Chatbot Types
Type | Strengths | Weaknesses |
---|---|---|
Rule-Based | Fast, predictable responses, easy to implement, low maintenance. | Limited adaptability, struggles with complex or nuanced requests, lacks learning capabilities. |
Machine Learning (ML) | High adaptability, learns from interactions, can handle complex tasks, more natural conversation. | Requires significant data for training, potentially expensive to develop and maintain, can be prone to biases. |
Hybrid | Combines strengths of rule-based and ML approaches, offers balance between speed and adaptability. | Implementation can be more complex, maintenance may require specialized expertise. |
Illustrative Examples of Exceptional Chatbots
Beyond the theoretical framework of defining “best” chatbots, understanding practical implementations is crucial. This section dives into concrete examples, examining their unique features, design choices, and real-world applications. We’ll explore how these chatbots excel in user engagement and satisfaction.Exceptional chatbots are not just sophisticated conversational agents; they are tools that solve real-world problems, seamlessly integrating into various aspects of our lives.
Their effectiveness hinges on careful design, sophisticated algorithms, and a deep understanding of user needs.
Looking at the best chatbot examples, you quickly realize how they’re changing how businesses interact with customers. These chatbots are increasingly sophisticated, but understanding how to drive traffic to them is key. For example, Facebook Dynamic Ads are a powerful tool for reaching the right people, and this guide on facebook dynamic ads 101 explains how to use them effectively.
Ultimately, the best chatbots are often those that integrate seamlessly with targeted advertising campaigns, making them a truly valuable addition to any business strategy.
Prominent Chatbot Examples
Several notable chatbots have emerged, each with its own strengths and unique capabilities. These examples demonstrate the potential and the challenges of chatbot development.
- Google Assistant: Google Assistant is a ubiquitous example of a task-oriented chatbot integrated into various devices, from smartphones to smart speakers. Its strength lies in its comprehensive access to Google’s vast knowledge base. This allows it to answer a wide array of questions, set reminders, control smart home devices, and perform various actions through voice commands. The design choices behind Google Assistant emphasize seamless integration with other Google services and a natural language processing (NLP) engine that understands user intent effectively.
Google Assistant’s extensive use cases range from simple information retrieval to complex tasks like scheduling appointments, and its impressive user engagement stems from its consistent accuracy and reliability.
- Kami: Developed by OpenAI, Kami represents a significant advancement in conversational AI. Its strength lies in its ability to generate human-like text, engage in creative writing, and translate languages. The design choices focus on large language models (LLMs) trained on massive datasets of text and code. Kami excels in generating creative content, answering complex questions, and providing insightful responses, demonstrating impressive user engagement and satisfaction through the quality of its responses.
Looking at the best chatbot examples, it’s clear they’re not just fancy tech; they’re powerful tools. Understanding the strengths and weaknesses of these chatbots, like a thorough SWOT analysis, is crucial. A good example of this is the right way conduct swot analysis here , helps identify the key factors driving their success. Ultimately, this analysis is just one more step towards developing even better chatbots in the future.
The use cases for Kami are diverse, from writing code to crafting poems, showing the vast potential of this technology.
- Siri: Siri, developed by Apple, is another prominent example of a voice-activated personal assistant. Its strength is its integration with Apple’s ecosystem. Siri utilizes NLP to understand user commands and perform tasks like making calls, sending messages, setting reminders, and accessing information. The design choices prioritize user experience and seamless integration within the Apple ecosystem. Siri’s use cases include daily tasks, information access, and controlling compatible devices, with a focus on user satisfaction through ease of use and efficiency.
Comparative Analysis of Chatbot Features
To highlight the diverse capabilities of these chatbots, a comparative table is presented below.
Name | Application | Key Features | User Ratings |
---|---|---|---|
Google Assistant | Personal assistant, information retrieval, smart home control | Voice-activated, access to Google’s knowledge graph, integrated with various services | High (consistent accuracy and reliability) |
Kami | Conversational AI, creative writing, code generation, language translation | Large language model, human-like text generation, extensive dataset training | High (quality of responses) |
Siri | Personal assistant, task management, information access | Voice-activated, integration with Apple ecosystem, NLP-driven | High (ease of use and efficiency) |
Analyzing Strengths and Weaknesses
Examining the strengths and weaknesses of exemplary chatbots provides crucial insights into the current state of chatbot technology and the path forward for improvement. Understanding their limitations is just as important as recognizing their capabilities, as it reveals areas where further development is needed. This analysis delves into the core strengths and weaknesses of different chatbot examples, highlighting key factors that contribute to their success or failure.
Core Strengths of Exemplary Chatbots
Exceptional chatbots possess several key strengths that contribute to their effectiveness. These include the ability to understand and respond to complex natural language queries, efficiently retrieve and process information, and personalize interactions to meet individual user needs. Advanced chatbots can handle a wide range of tasks, from simple customer service requests to complex technical support issues. Their ability to learn from interactions and adapt to user preferences enhances their effectiveness over time.
- Natural Language Understanding (NLU): Many exemplary chatbots excel at understanding nuanced language, including slang, colloquialisms, and complex sentence structures. This ability to interpret intent accurately is critical for providing appropriate and relevant responses. Examples include chatbots that can interpret customer frustration or identify specific technical issues based on user input.
- Information Retrieval and Processing: The ability to quickly and accurately access and process information from diverse sources is a crucial strength. This often involves utilizing vast databases, knowledge graphs, and external APIs. Effective chatbots can not only answer questions but also synthesize information to offer comprehensive solutions.
- Personalization and Adaptability: Modern chatbots are increasingly capable of tailoring their interactions to individual user preferences. This includes learning user history, preferences, and past interactions to provide more relevant and personalized responses. The use of machine learning algorithms plays a key role in this adaptability.
Limitations and Potential Areas for Improvement
While exemplary chatbots demonstrate significant strengths, they also face limitations. One key area is the accuracy and consistency of responses. Chatbots may struggle with ambiguous or unusual queries, leading to incorrect or irrelevant responses. Another challenge is maintaining context across multiple interactions. Maintaining consistent and accurate information retrieval and processing across various sessions remains a significant hurdle.
Finally, ethical considerations, such as bias in training data and potential for misuse, must be addressed in the development of these systems.
- Handling Ambiguity and Unusual Input: Chatbots often struggle with unexpected or unusual input, including slang, typos, or poorly formulated questions. This can lead to inaccurate or unhelpful responses. Training data often cannot anticipate every possible variation in user input, which leads to limitations in handling such situations.
- Contextual Awareness and Memory: Maintaining context across multiple interactions is a significant challenge. Chatbots may forget previous exchanges or misinterpret subsequent requests due to a lack of sustained memory. This can lead to inconsistencies and a fragmented user experience.
- Ethical Considerations: The training data used to develop chatbots can reflect existing societal biases. This can lead to unfair or discriminatory outcomes in certain situations. The potential for misuse and the need for robust security measures are crucial factors to consider when developing these systems.
Factors Contributing to Chatbot Success or Failure
Several factors play a crucial role in determining the success or failure of a chatbot. These include the quality and comprehensiveness of the training data, the sophistication of the underlying algorithms, the ease of use for the end-user, and the integration with other systems. The ability to handle diverse inputs, adapt to user preferences, and maintain context throughout interactions are essential for success.
Comparison of Strengths and Weaknesses Across Examples
Chatbot | Strength 1 | Strength 2 | Weakness 1 | Weakness 2 |
---|---|---|---|---|
Example Chatbot 1 | Excellent NLU for specific domains | Fast information retrieval | Struggles with open-ended questions | Limited context maintenance |
Example Chatbot 2 | Highly personalized interactions | Adaptable to user preferences | Potential for bias in responses | Difficulty handling complex queries |
Example Chatbot 3 | Comprehensive knowledge base | Handles multiple languages | Slow response times in complex scenarios | Limited ability to learn from user feedback |
User Experience and Interface Design
The success of a chatbot hinges not just on its functionality but also on how easily and pleasantly users interact with it. A well-designed user experience (UX) fosters user satisfaction, encourages engagement, and ultimately drives adoption. This crucial aspect goes beyond simple functionality, encompassing the overall impression and ease of use the chatbot provides.A user-friendly chatbot interface impacts user satisfaction and engagement significantly.
Users are more likely to continue using a chatbot if they find the interaction intuitive and straightforward. A positive experience encourages repeat use, builds trust, and potentially converts users into loyal customers or clients. Conversely, a poorly designed interface can lead to frustration, abandonment, and negative brand perception.
Importance of User-Friendly Interface
A chatbot’s interface plays a pivotal role in determining its effectiveness. A user-friendly design ensures that users can effortlessly navigate the interaction, understand the chatbot’s capabilities, and receive the desired information or assistance without undue effort. This translates directly into user satisfaction and positive engagement. The ability to quickly and easily find answers, initiate actions, and comprehend the chatbot’s responses are critical for a seamless experience.
Examples of Effective Chatbot Interfaces
Several notable chatbots demonstrate best practices in user interface design. Their success stems from clear communication, intuitive navigation, and tailored interaction styles. Examples like the chatbots employed by major e-commerce platforms often provide a streamlined shopping experience, while customer service chatbots excel in offering quick and helpful support. The interface design directly influences the user’s perception of the chatbot’s intelligence and usefulness.
Designing an Intuitive Chatbot Interface
Designing an intuitive chatbot interface requires careful consideration of various factors. Key elements include clear prompts, concise responses, and a consistent conversational flow. Users should readily understand the chatbot’s capabilities and how to initiate interactions. Visual cues, such as buttons, icons, and formatting, should support and enhance understanding. Using natural language processing (NLP) to enable natural and human-like conversation is also essential for a positive user experience.
UX Elements of Notable Chatbots
Chatbot | Interface Features | User Feedback | Design Principles |
---|---|---|---|
E-commerce Chatbot (Example: Amazon) | Clear product listings, simple ordering process, quick response to queries, integrated with other platform features. | Positive feedback regarding ease of use, speed, and efficiency. Users appreciate the ability to quickly find information and complete tasks. | Simplicity, efficiency, and integration with existing platform infrastructure. Prioritization of quick task completion. |
Customer Service Chatbot (Example: Bank) | Clear navigation for different support options, concise FAQs, and options for escalation to human agents. Use of visuals to guide the user. | Users appreciate the ability to quickly find solutions to common problems and the availability of human assistance. Complaints often related to unclear pathways or technical issues. | Clarity, accessibility, and options for escalation. Emphasis on providing solutions directly. |
Social Media Chatbot (Example: Facebook Messenger) | Conversational style, intuitive navigation through menus, ability to send and receive images/videos. | Positive feedback regarding the conversational flow and ability to interact with the bot in a natural way. Some users noted difficulty with complex queries. | Natural language processing, visual elements, and a focus on human-like conversation. |
Technical Aspects and Capabilities: The Best Chatbots Examples
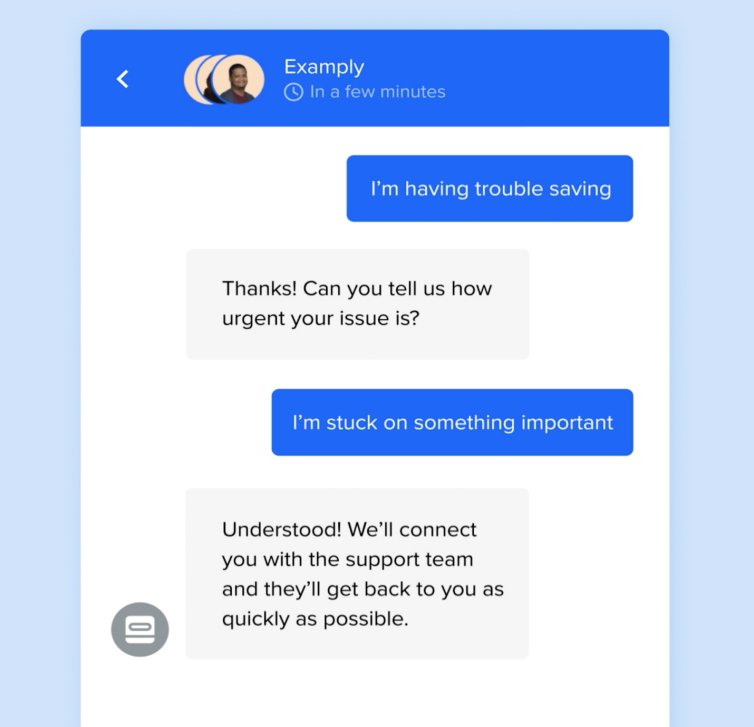
The “best” chatbots aren’t just clever; they’re sophisticated engines powered by cutting-edge technology. Understanding the underlying technical components illuminates the secrets behind their impressive abilities. This section delves into the key technical elements, focusing on natural language processing (NLP) and machine learning (ML), to expose how these technologies contribute to the overall functionality of these advanced conversational agents. We will also look at concrete examples to illustrate these concepts.The power of modern chatbots hinges on a complex interplay of NLP and ML techniques.
These technologies enable the chatbot to understand and respond to human language with increasing accuracy and nuance, mimicking human conversation. The more sophisticated the NLP and ML, the more realistic and human-like the chatbot’s interactions become.
Key Technical Components
The foundation of a sophisticated chatbot rests on several key components. These include sophisticated natural language understanding (NLU) modules, robust dialogue management systems, and efficient knowledge representation methods. These elements work together to enable the chatbot to comprehend, interpret, and respond to user input.
- Natural Language Processing (NLP): NLP is the backbone of chatbot communication. It encompasses techniques to enable computers to understand, interpret, and generate human language. This involves tasks such as tokenization (breaking down text into words), part-of-speech tagging, named entity recognition, and sentiment analysis. These tasks allow the chatbot to grasp the meaning and intent behind user queries.
- Machine Learning (ML): ML algorithms form the core of a chatbot’s ability to learn and adapt. Supervised learning models, trained on vast datasets of conversations, enable the chatbot to learn patterns in human language and improve its responses over time. Reinforcement learning algorithms can fine-tune the chatbot’s interactions with users, making them more effective and engaging. Unsupervised learning techniques can also discover hidden patterns in user data to improve overall performance.
- Dialogue Management: This component orchestrates the conversation flow, ensuring a coherent and meaningful exchange. It decides which responses to generate, tracks the context of the conversation, and manages potential ambiguities. Sophisticated dialogue management systems are crucial for maintaining a fluid and natural-sounding conversation.
Natural Language Processing (NLP) Techniques
NLP techniques are crucial for understanding and generating human-like text. These techniques allow the chatbot to process and interpret user input, identify key entities, and respond appropriately. Examples include sentiment analysis (detecting the emotional tone of a message) and intent recognition (determining the user’s goal).
Ever wondered about the coolest chatbot examples out there? They’re amazing, right? But sometimes, you need a quick video edit for Instagram. Fortunately, there are tons of great options for video editing apps, both free and paid, like the best video editing apps for instagram free paid apps. Once you’ve got your video looking sharp, you can return to exploring those amazing chatbot examples again!
- Tokenization: Breaking down text into individual words or units (tokens). This is a fundamental step in NLP, enabling further analysis of the text.
- Part-of-Speech Tagging: Identifying the grammatical role of each word in a sentence (e.g., noun, verb, adjective). This helps in understanding the structure and meaning of the sentence.
- Named Entity Recognition: Identifying and classifying named entities (e.g., people, organizations, locations). This is crucial for extracting specific information from user input.
Machine Learning (ML) Workflow
The ML workflow for chatbots involves training the chatbot on a large dataset of conversations. This data is used to build a model that can predict the most appropriate response to a given input. This process involves iterative refinement and improvement.
- Data Collection: Gathering a large dataset of conversations. This data can come from various sources, including previous interactions with users, public forums, and dedicated datasets.
- Model Training: Using the collected data to train an ML model. This involves feeding the data to an algorithm that learns patterns and relationships between inputs and outputs. Different algorithms are employed depending on the complexity of the task, like recurrent neural networks (RNNs) or transformers.
- Evaluation: Assessing the model’s performance using metrics such as accuracy and precision. This allows for identification of areas needing improvement.
- Deployment: Integrating the trained model into the chatbot system for real-time use.
Example: A Customer Support Chatbot
A customer support chatbot might use a transformer-based model for NLP. The model would be trained on a massive dataset of customer support interactions, including questions, answers, and the context of each conversation. This training enables the chatbot to understand customer inquiries and provide accurate solutions. This type of chatbot would employ dialogue management to handle complex inquiries and provide a seamless user experience.
Future Trends and Developments
Chatbots are rapidly evolving, moving beyond simple rule-based systems to more sophisticated AI-powered models. This evolution is driven by advancements in natural language processing (NLP), machine learning (ML), and deep learning (DL). The future of chatbots promises a wider range of applications and a more seamless user experience.The next generation of chatbots will likely exhibit greater adaptability, context awareness, and even emotional intelligence.
These improvements will allow them to handle more complex tasks and interact with users in a more natural and human-like manner.
Emerging Trends in Chatbot Technology
Advancements in NLP, particularly in areas like semantic understanding and contextual awareness, are significantly impacting chatbot capabilities. These advancements allow chatbots to grasp nuances in language and understand the context of a conversation, leading to more accurate and relevant responses. This capability is crucial for tasks like complex customer service interactions, where understanding the user’s specific needs and past interactions is vital.
Advancements in Natural Language Processing (NLP)
Sophisticated NLP models, such as transformers, are pushing the boundaries of conversational AI. These models can understand and generate human language with remarkable accuracy, enabling more fluid and natural conversations. Examples include the ability to answer complex questions, summarize information, and even generate creative text formats.
Integration with Other Technologies
The integration of chatbots with other technologies, such as augmented reality (AR) and virtual reality (VR), is a key future trend. This integration can enhance user experiences by providing more immersive and interactive interactions. For instance, a chatbot integrated with AR could guide users through a product demonstration or provide real-time support during a virtual event.
Personalized and Adaptive Chatbots, The best chatbots examples
Future chatbots will likely be more personalized and adaptive. These chatbots will learn user preferences and behaviors over time, tailoring their responses and interactions accordingly. This personalization will result in a more engaging and efficient user experience. Imagine a chatbot that learns your preferred shopping style and recommends products tailored to your needs.
Impact of Emerging Technologies on Chatbot Capabilities
The impact of emerging technologies on chatbots is profound. Advancements in AI, specifically in areas like deep learning and reinforcement learning, are improving the ability of chatbots to understand context, adapt to different user styles, and provide more comprehensive responses. These developments will lead to more sophisticated and efficient conversational interfaces in various applications.
Table of Future Trends and Potential Impact
Trend | Description | Potential Impact |
---|---|---|
Advancements in NLP | Improved semantic understanding, contextual awareness, and generation of human-like text | More accurate and relevant responses, enabling more complex tasks like summarization and creative text generation |
Integration with other technologies (AR/VR) | Combining chatbots with immersive technologies to enhance user experiences | More engaging and interactive user experiences in various applications like product demonstrations and virtual events |
Personalized and Adaptive Chatbots | Chatbots that learn user preferences and behaviors to tailor interactions | More efficient and engaging user experience, improved recommendations, and enhanced customer satisfaction |
Evolution of AI Models | Development of more sophisticated AI models (deep learning, reinforcement learning) | Improved understanding of context, adaptability to different user styles, and more comprehensive responses |
Closing Summary
In conclusion, the best chatbots examples represent a significant leap forward in AI technology. Their ability to seamlessly integrate into various aspects of our lives highlights the potential for further advancements. We’ve explored the design choices, technical innovations, and user experiences that contribute to their success, emphasizing the importance of user-centric design and advanced technology. The future of chatbots is bright, and this exploration has provided a solid foundation for understanding this exciting evolution.