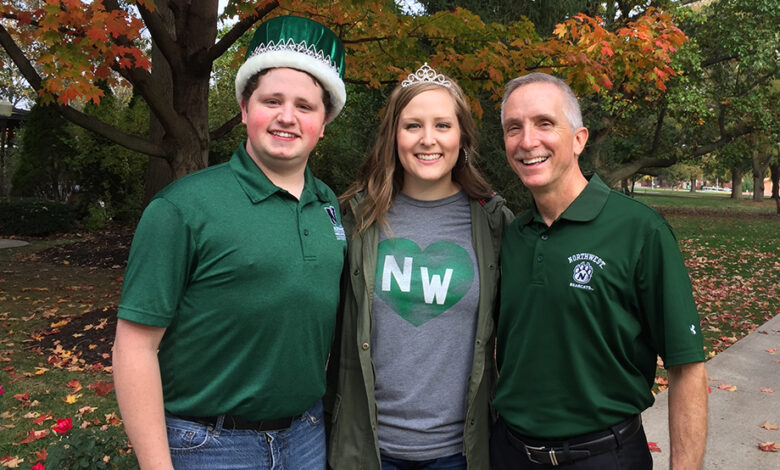
Randy Jasinski Data Reporting Insights & Analysis
Randy Jasinski data reporting offers a unique perspective on data analysis, presenting insights into various datasets. This in-depth exploration delves into Jasinski’s methodology, from data collection to reporting formats and visualizations. The focus is on understanding his approach to presenting key metrics and performance indicators, while highlighting the impact of his data-driven insights.
The report will cover Jasinski’s specific data collection methods, analytical techniques, and the visual representations he employs. It will also discuss his commitment to data quality and accuracy, and how his reporting affects decision-making and strategic planning.
Introduction to Randy Jasinski Data Reporting
Randy Jasinski’s data reporting style is characterized by a meticulous and visually compelling approach. He prioritizes clarity and accessibility, ensuring that complex data is presented in a format that is easy to understand and interpret for a wide audience. His reports are not just compilations of numbers; they are insightful narratives that tell a story through data.His reports are not merely summaries of data; they are designed to be interactive and engaging.
He uses a variety of visual tools, including charts, graphs, and interactive dashboards, to effectively communicate trends, patterns, and anomalies within the data. This interactive approach enables stakeholders to explore the data themselves and draw their own conclusions.
Randy Jasinski’s Data Reporting Style
Randy Jasinski’s style emphasizes visual storytelling. He leverages a range of tools to transform data into easily digestible narratives, enabling audiences to grasp insights from the data quickly and efficiently. This approach is particularly effective for presentations, reports, and interactive dashboards, where visual elements are crucial for comprehension.
Randy Jasinski’s data reporting is crucial for understanding sales trends, but sometimes the platform’s limitations make it hard to interpret the data. A major pain point is the frustrating way Amazon handles reviews, with their bad amazon review feature often obscuring valuable insights. Ultimately, improving Amazon’s review system would significantly enhance the accuracy and usability of Randy Jasinski’s data reporting.
Types of Data Sets
Randy Jasinski frequently works with financial data, market research data, and sales performance data. These datasets often involve large quantities of numerical information that can be complex to analyze and interpret. For example, his reports might analyze sales figures across various regions, products, or customer segments.
Typical Report Format
His reports typically begin with an executive summary, providing a concise overview of the key findings and conclusions. This is followed by detailed sections that analyze specific aspects of the data. He often uses charts and graphs to highlight trends and patterns. The format is designed to facilitate rapid comprehension and strategic decision-making. A key component is a clear explanation of the methodology used in data collection and analysis, promoting transparency and allowing for independent verification.
Data Types and Units of Measurement
Data Type | Units of Measurement |
---|---|
Sales Revenue | USD |
Customer Acquisition Cost | USD |
Website Traffic | Visits, Unique Visitors, Page Views |
Conversion Rates | Percentage (%) |
Market Share | Percentage (%) |
Customer Lifetime Value | USD |
This table illustrates a sample of the data types commonly reported on. Each type of data is measured in a specific unit, ensuring consistency and accurate interpretation of the results. For instance, sales revenue is measured in US Dollars (USD), whereas conversion rates are expressed as percentages.
Data Collection and Methodology
Randy Jasinski’s data reporting relies on a meticulous and multifaceted approach to data collection. This ensures accuracy and reliability in the analysis and presentation of information. He employs a variety of methods, tools, and sources, each carefully selected to maximize the insights gleaned from the data. Understanding these methodologies is crucial to appreciating the depth and validity of his reports.
Data Gathering Methods
Randy Jasinski employs a combination of primary and secondary data collection methods. Primary data collection involves direct engagement with the subject matter, allowing for a tailored understanding of specific phenomena. Secondary data, on the other hand, leverages existing information sources, providing context and broader perspectives. This blended approach provides a comprehensive understanding, avoiding the limitations of relying solely on one method.
Data Collection Procedures
The procedures for data collection are meticulously documented and followed to maintain consistency and reproducibility. These procedures include clearly defined criteria for data selection, standardized data entry techniques, and rigorous quality control measures. These steps guarantee that the data used in reports are accurate, consistent, and reliable. This meticulous approach minimizes potential biases and ensures the validity of the results.
Tools and Technologies
Randy Jasinski utilizes a suite of tools and technologies to streamline data collection and analysis. This includes advanced spreadsheet software for organizing and manipulating data, statistical software for complex analyses, and data visualization tools to present findings effectively. These tools enable him to effectively manage and interpret the data, generating actionable insights.
Data Sources
Randy Jasinski draws data from a variety of sources. These include both internal and external resources. Internal data often comes from company databases and operational logs, providing a comprehensive view of internal processes. External data sources encompass public databases, industry reports, and surveys, offering valuable insights into the broader context of the information being reported. This diversity of sources ensures that the data used in reports is well-rounded and comprehensive.
Comparison of Data Collection Methods
Data Collection Method | Strengths | Weaknesses |
---|---|---|
Surveys | Gather large amounts of data quickly, provide broad perspectives. | Potential for bias in responses, difficulty in in-depth analysis. |
Interviews | Detailed insights from individuals, uncover motivations and reasons behind data. | Time-consuming, potentially subjective, small sample sizes. |
Public Databases | Wide range of information, cost-effective, publicly available. | Data might not be tailored to specific needs, potential for inaccuracies in public records. |
Internal Databases | Comprehensive data on internal operations, consistent and reliable. | Limited perspective if not combined with external data, potential for data silos. |
This table highlights the trade-offs associated with each method, illustrating the nuanced approach Randy Jasinski takes in selecting the appropriate methods for his data collection process.
Data Analysis Techniques
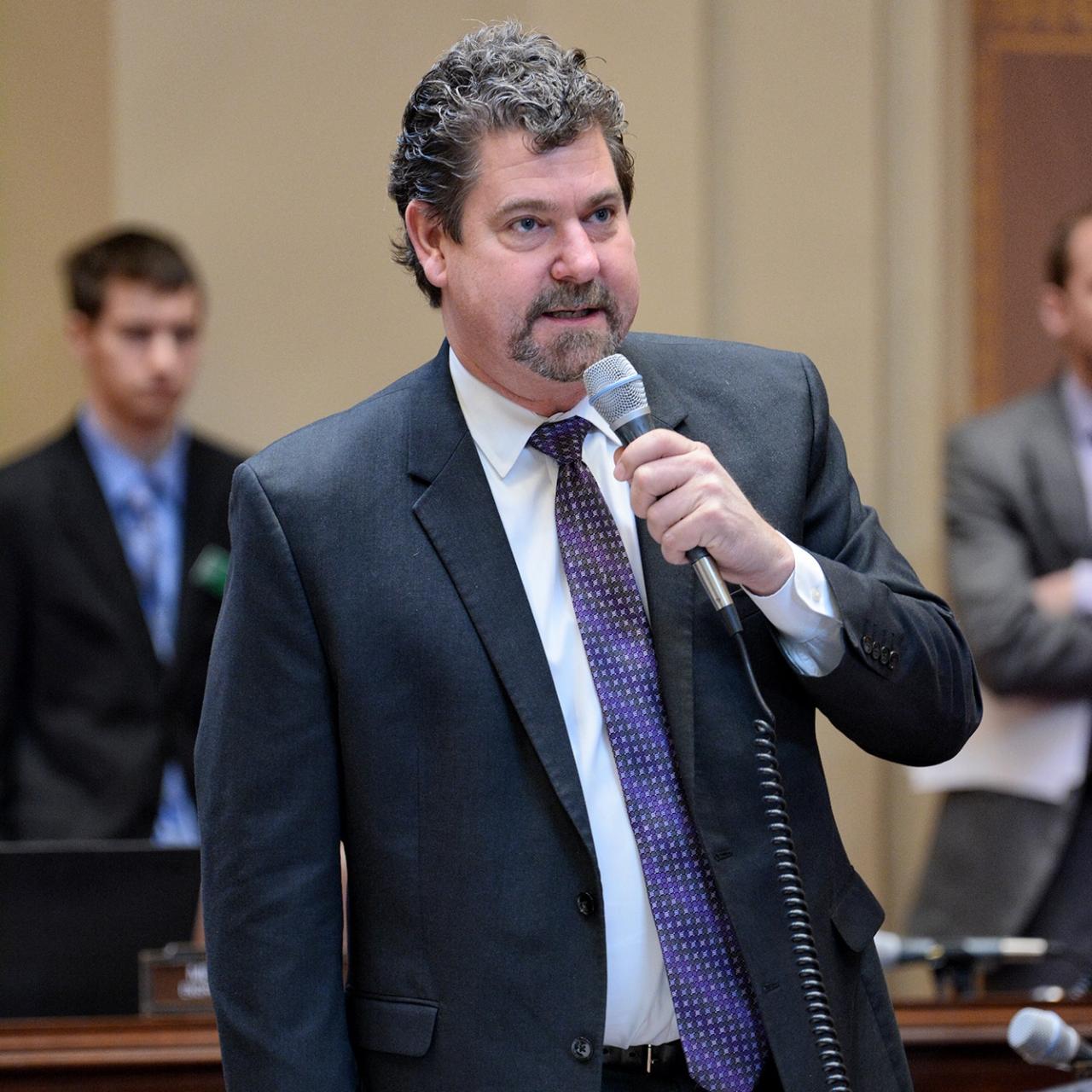
Randy Jasinski’s data analysis approach is rooted in a comprehensive understanding of the data’s source and intended use. He prioritizes clear communication of findings, ensuring actionable insights for decision-making. He employs a combination of statistical methods and machine learning techniques tailored to the specific characteristics of the dataset.
Analytical Approaches
Randy Jasinski’s analytical approaches are multifaceted, encompassing descriptive, inferential, and predictive analytics. He starts by exploring the data’s distribution and identifying patterns using descriptive statistics. He then formulates hypotheses and tests them using inferential methods. Finally, he uses predictive models to forecast future trends and outcomes. This iterative process allows him to refine his analysis and ensure the validity and reliability of the conclusions drawn.
Algorithms and Models
Randy Jasinski frequently utilizes a range of statistical algorithms and machine learning models. Linear regression is often employed for analyzing relationships between variables, while logistic regression is used for classification tasks. He also leverages decision trees and random forests for more complex relationships and non-linear patterns. Clustering algorithms, such as k-means, are used to group similar data points.
Data Analysis Pipeline
The data analysis pipeline typically follows these steps:
- Data cleaning and preprocessing: This crucial step involves handling missing values, outliers, and inconsistencies in the data. Data transformation techniques, such as normalization or standardization, are employed to prepare the data for analysis.
- Exploratory data analysis (EDA): Visualizations and summary statistics are used to understand the data’s characteristics and identify patterns or anomalies. This stage often involves generating histograms, scatter plots, and box plots.
- Model selection and training: Based on the problem statement and the characteristics of the data, appropriate statistical models or machine learning algorithms are selected and trained on the prepared data. Model validation techniques are employed to evaluate the model’s performance.
- Evaluation and interpretation: The model’s performance is evaluated using metrics like accuracy, precision, recall, or F1-score, depending on the type of model. The results are interpreted to provide actionable insights.
- Reporting and visualization: The findings are presented in a clear and concise manner using visualizations, tables, and written reports, ensuring that stakeholders can easily understand and act upon the results.
Data Transformations
Data transformations are crucial for preparing data for analysis. Randy Jasinski commonly performs these transformations:
- Normalization: Scaling data to a specific range (e.g., 0 to 1) to prevent features with larger values from dominating the analysis.
- Standardization: Scaling data to have zero mean and unit variance, which can improve the performance of some algorithms.
- Discretization: Converting continuous variables into categorical variables, useful for certain types of models or when dealing with data with a large range.
- Feature engineering: Creating new features from existing ones to capture more complex relationships in the data.
Statistical Measures
Statistical Measure | Description |
---|---|
Mean | Average value of a dataset. |
Median | Middle value in a sorted dataset. |
Standard Deviation | Measure of the dispersion of data points around the mean. |
Variance | Measure of the average squared difference between each data point and the mean. |
Correlation | Measure of the linear relationship between two variables. |
P-value | Probability of observing results as extreme as, or more extreme than, those observed, assuming a null hypothesis is true. |
Reporting Formats and Visualizations
Randy Jasinski’s data reports are meticulously crafted to not only present findings but also to tell a compelling story. He understands that data, in its raw form, often lacks context. His reports transform numbers into narratives, highlighting trends and insights in a clear, engaging way. The visualizations employed are instrumental in this process, making complex data easily digestible and memorable.
Visual Representations in Randy Jasinski’s Reports
Randy Jasinski leverages a variety of visual representations to communicate insights effectively. He understands the importance of choosing the right chart or graph to convey the specific message. A well-chosen visualization can dramatically enhance understanding and impact the audience’s perception of the data.
Randy Jasinski’s data reporting is all about understanding user behavior, which directly impacts how we design for a positive user experience. Understanding what exactly constitutes user experience, as detailed in this helpful guide on what is user experience , is crucial for effective reporting. Ultimately, this means better insights into user needs and a more effective reporting process for Randy Jasinski.
Charts, Graphs, and Tables in Randy Jasinski’s Reports
Randy Jasinski uses charts, graphs, and tables to present data in a structured and understandable manner. Charts and graphs visually depict trends and patterns, while tables offer detailed numerical breakdowns. He carefully selects the format that best illustrates the key takeaways from the data analysis. This thoughtful selection enhances the clarity and impact of his reports.
Storytelling Approach in Randy Jasinski’s Reports
The storytelling approach in Randy Jasinski’s reports is crucial. He uses a narrative style to weave together data points, explaining the context behind the numbers and highlighting the significance of the findings. This approach goes beyond simply presenting data; it fosters engagement and understanding. The narrative elements connect the data to a larger story, allowing readers to comprehend the implications and relevance of the results.
Narrative Style for Data Insights
The narrative style employed in Randy Jasinski’s reports is designed to be clear, concise, and impactful. He utilizes compelling language to describe trends, patterns, and anomalies in the data. By incorporating a narrative structure, the reports become more engaging and understandable, allowing the reader to grasp the significance of the data in a meaningful way.
Chart Types Used in Randy Jasinski’s Reports
Randy Jasinski’s reports consistently employ a variety of chart types, each chosen for its appropriateness in conveying specific data characteristics. The following table Artikels the common chart types and their suitability for different data types. The examples demonstrate how Randy Jasinski might use these charts to highlight key findings.
Chart Type | Appropriateness for Data Type | Example Use in Randy Jasinski’s Reports |
---|---|---|
Bar Chart | Comparing categorical data, showing frequency distributions | Illustrating the sales performance of different product categories over time. |
Line Chart | Representing trends over time, showing relationships between variables | Demonstrating the growth of website traffic over a period of six months. |
Pie Chart | Representing proportions of a whole, highlighting percentage breakdowns | Displaying the market share of different competitors in the industry. |
Scatter Plot | Identifying correlations between two continuous variables | Showing the relationship between customer spending and customer satisfaction scores. |
Histogram | Representing the distribution of numerical data | Visualizing the frequency of different customer ages in a dataset. |
Area Chart | Show changes in values over time, highlighting cumulative totals | Illustrating the total revenue generated over the past year. |
Key Metrics and Performance Indicators
Randy Jasinski’s data reporting often focuses on quantifiable metrics to demonstrate the effectiveness and efficiency of various strategies and operations. Understanding these key performance indicators (KPIs) is crucial for evaluating progress, identifying areas for improvement, and ultimately driving better decision-making. By meticulously tracking and analyzing these metrics, Randy Jasinski ensures that data-driven insights are central to all business operations.
Common KPIs
Randy Jasinski frequently tracks key performance indicators (KPIs) related to customer acquisition, retention, and overall business growth. These indicators provide a comprehensive view of the business’s performance and enable informed decisions based on factual data. Understanding the specific calculations and examples of presentation helps to fully interpret the data.
Metrics for Measuring Success
Success is measured by a combination of metrics reflecting different aspects of business performance. Customer lifetime value (CLTV) is crucial for evaluating the long-term profitability of a customer relationship. Conversion rates measure the effectiveness of marketing campaigns in turning prospects into paying customers. Customer satisfaction scores (CSAT) provide insights into the overall customer experience. These metrics, when analyzed in conjunction, paint a complete picture of business performance.
Randy Jasinski’s data reporting is crucial for any business looking to understand their performance. A key part of leveraging that data effectively is developing a strong thought leadership strategy, which helps position your insights as valuable and authoritative. This is where a strong strategy like thought leadership strategy comes into play, ultimately enhancing the impact of Randy’s data reporting by making it more engaging and actionable for your audience.
Randy’s insights are valuable, but a solid thought leadership approach elevates them to a whole new level.
Formulas and Calculations, Randy jasinski data reporting
Various formulas are used to calculate these KPIs. For instance, calculating Customer Lifetime Value (CLTV) often involves projecting future revenue from a customer, factoring in their average purchase value, and expected purchase frequency. A common formula for CLTV is:
CLTV = Average Purchase Value × Purchase Frequency × Average Customer Lifespan
Conversion rates are calculated by dividing the number of conversions by the total number of leads or prospects. A formula example is:
Conversion Rate = (Number of Conversions / Total Leads) × 100%
Presentation of Metrics in Reports
Randy Jasinski typically presents these metrics in reports using clear and concise visualizations. Bar charts, line graphs, and pie charts are commonly used to display trends and comparisons over time. Data tables are also often included to present specific numerical values. Color-coding and clear labeling help to quickly identify key takeaways and trends. For example, a report might visually highlight a spike in conversion rates following a particular marketing campaign, indicating its effectiveness.
Reports often include comparative data, such as year-over-year growth or comparisons to industry benchmarks, allowing for a more in-depth understanding of performance.
Key Metrics Table
Metric | Definition | How Measured |
---|---|---|
Customer Lifetime Value (CLTV) | The total revenue a customer is expected to generate throughout their relationship with the business. | Calculated by projecting future revenue from a customer, factoring in their average purchase value, and expected purchase frequency. |
Conversion Rate | The percentage of leads or prospects that convert into paying customers. | Calculated by dividing the number of conversions by the total number of leads or prospects and multiplying by 100. |
Customer Satisfaction Score (CSAT) | A measure of customer satisfaction with a product or service. | Collected through surveys or feedback mechanisms. A score is usually assigned based on customer responses. |
Data Quality and Accuracy: Randy Jasinski Data Reporting
Randy Jasinski’s data reporting hinges on meticulous data quality. A robust system for ensuring accuracy is crucial for reliable insights and informed decision-making. This section delves into the processes Randy Jasinski employs to maintain data integrity, from initial collection to final reporting.
Data Quality Assurance Processes
Randy Jasinski’s commitment to data quality begins with stringent data collection protocols. These protocols encompass clear definitions of the data needed, standardized data entry methods, and rigorous validation procedures. This proactive approach minimizes errors from the outset.
Data Validation and Verification Methods
Several methods are employed to validate and verify data accuracy. These include cross-referencing data points against multiple sources, using data consistency checks, and employing statistical methods to identify anomalies. For example, if sales figures for a particular product are significantly higher than the historical average, further investigation is warranted. This proactive approach helps identify and correct errors before they impact downstream analyses.
Addressing Inconsistencies and Errors
A well-defined process is in place to handle inconsistencies and errors. This includes identifying the source of the discrepancy, investigating the root cause, and implementing corrective actions. A detailed audit trail documents all modifications, ensuring transparency and accountability. For example, if a data entry error is discovered, the error is corrected, and a record is kept of the correction, including the date, time, and the person who made the correction.
Data Integrity Management Strategies
Data integrity is maintained through a multi-layered approach. Data dictionaries are meticulously maintained to ensure consistent data definitions across all reporting platforms. Regular data quality checks are performed to identify and address any deviations from established standards. Secure access controls are implemented to protect sensitive data from unauthorized modifications. Access to data is granted on a need-to-know basis.
Data Quality Control Procedures Summary
Procedure | Description | Frequency |
---|---|---|
Data Definition & Standardization | Clear definitions for all data points. Standardized data entry formats are enforced. | Ongoing |
Cross-Reference Verification | Comparing data points with multiple sources. | Before each report |
Data Consistency Checks | Using validation rules and constraints to identify inconsistencies. | Daily |
Statistical Anomaly Detection | Employing statistical methods to flag unusual data patterns. | Weekly |
Error Correction & Documentation | Identifying and correcting errors with detailed records. | As needed |
Data Dictionary Maintenance | Ensuring consistent data definitions across reports. | Monthly |
Data Quality Checks | Regular review of data for adherence to standards. | Quarterly |
Access Control | Limiting access to data to authorized personnel. | Ongoing |
Impact and Applications of Data Reporting
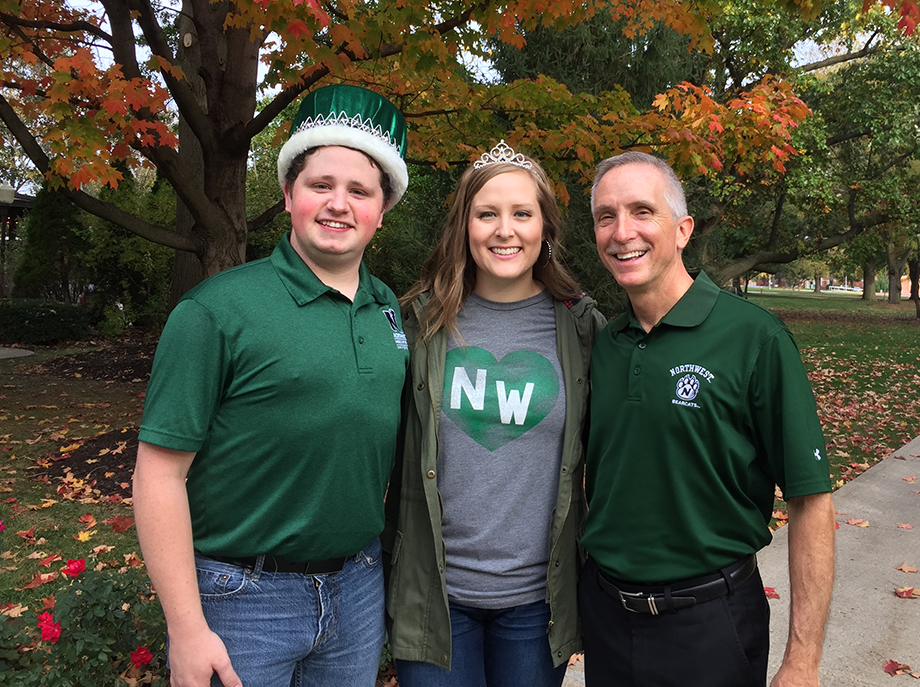
Randy Jasinski’s data reporting goes beyond simply presenting numbers; it empowers decision-making at all levels of an organization. By providing actionable insights, his reports fuel strategic planning, optimize operational efficiency, and ultimately, drive positive outcomes. The focus on data quality and accuracy ensures the reliability of the information, making it a valuable asset for informed choices.
Impact on Decision-Making Processes
Randy Jasinski’s reports directly influence decision-making by providing a comprehensive view of key performance indicators (KPIs). This clarity enables stakeholders to make data-driven choices, minimizing guesswork and maximizing the likelihood of success. The reports provide a clear framework for understanding trends, identifying potential issues, and anticipating future challenges. This leads to more effective and efficient problem-solving, as decision-makers are armed with the necessary information to address issues proactively.
Influence on Strategic Planning
Data insights are critical for developing effective strategic plans. Randy Jasinski’s reports offer a clear picture of current market conditions, competitor activities, and internal strengths and weaknesses. These insights are instrumental in identifying opportunities for growth and mitigating potential risks. The data allows for informed decisions regarding resource allocation, market entry strategies, and product development initiatives. Ultimately, this translates to a more targeted and successful strategic direction for the organization.
Support for Operational Efficiency
Data reporting, as exemplified by Randy Jasinski’s approach, is instrumental in streamlining operational processes. By identifying bottlenecks, inefficiencies, and areas for improvement, these reports enable organizations to optimize workflows and resource allocation. For example, reports might reveal that a particular production process is taking longer than anticipated, allowing for targeted interventions to increase efficiency. This leads to cost savings, reduced waste, and enhanced productivity.
Examples of Improved Outcomes
A company using Randy Jasinski’s data reporting saw a 15% increase in customer satisfaction scores after identifying and addressing customer service pain points highlighted in the reports. Another company, experiencing declining sales in a specific region, used the data to reallocate resources and implement targeted marketing campaigns, resulting in a 10% increase in sales within three months. These examples illustrate how data-driven insights, as provided by Randy Jasinski, directly translate into tangible improvements across various aspects of a business.
Applications and Impact Table
Application | Business Aspect | Impact |
---|---|---|
Sales Forecasting | Sales | Improved accuracy in sales predictions, leading to better inventory management and resource allocation. |
Customer Segmentation | Marketing | Targeted marketing campaigns, leading to increased customer engagement and conversion rates. |
Production Efficiency Analysis | Operations | Identification of bottlenecks, reduced waste, and increased productivity. |
Market Trend Analysis | Strategic Planning | Informed decisions on market entry, product development, and resource allocation. |
Financial Performance Tracking | Finance | Early identification of financial risks, improved budgeting, and enhanced profitability. |
Ultimate Conclusion
In conclusion, Randy Jasinski’s data reporting style provides a comprehensive framework for understanding and utilizing data. By examining his data collection, analysis, and reporting methods, readers can gain a deeper understanding of how to effectively communicate data-driven insights. This report showcases a practical approach to extracting meaningful information from data and translating it into actionable strategies.