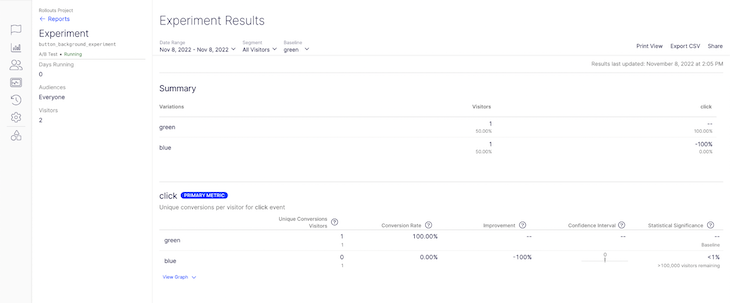
10 Best A/B Test Examples You Must Try Yourself
10 best a b test examples you must try yourself sets the stage for a deep dive into the world of A/B testing. We’ll explore real-world examples across various industries, from e-commerce to mobile apps and marketing campaigns. Discover how these tests can significantly impact your bottom line, boost conversions, and optimize user experience.
This comprehensive guide will cover everything from defining A/B testing and choosing the right metrics, to implementing effective tests, avoiding common pitfalls, and learning from successful case studies. Get ready to unlock the secrets of A/B testing and transform your strategies.
Introduction to A/B Testing
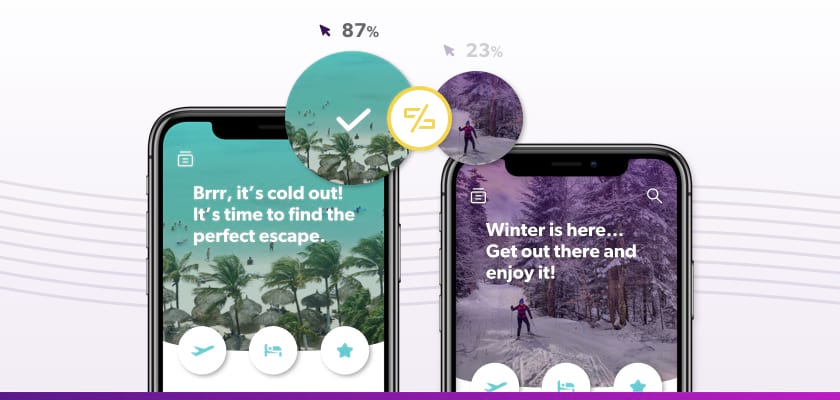
A/B testing is a controlled experiment methodology used to compare two versions of a variable (e.g., a webpage, an advertisement, a product feature) to determine which performs better. This systematic approach allows businesses and marketers to optimize their strategies and improve user experience and conversion rates. It’s a cornerstone of data-driven decision-making, particularly in the digital realm.Effective A/B testing hinges on a clear understanding of the desired outcome and a meticulous execution process.
By isolating the impact of specific changes, businesses can identify which modifications resonate best with their target audience, ultimately leading to enhanced user engagement and business growth. Crucial to this process is ensuring the experiment’s environment remains consistent for both versions, minimizing extraneous variables and maximizing the accuracy of results.
Key Principles of Effective A/B Testing
A/B testing relies on sound principles to yield meaningful results. These include:
- Defining a clear objective: Clearly identifying the desired outcome, such as increased click-through rates or higher conversion rates, is paramount. A well-defined objective guides the entire testing process and ensures that the results directly address the business goal.
- Controlled experiment design: Ensuring that only the variable being tested is altered while all other factors remain constant is crucial. This isolates the effect of the change, preventing inaccurate conclusions.
- Sufficient sample size: A large enough sample size is essential for statistically significant results. Insufficient data can lead to misleading conclusions and wasted resources.
- Random assignment: Users or customers are randomly assigned to either the control or variant group to eliminate bias and ensure that the groups are comparable.
- Statistical significance: Using statistical methods to determine if the observed difference between the control and variant groups is meaningful or simply due to random chance is critical.
Importance of Controlled Experiments in A/B Testing
Controlled experiments are the bedrock of A/B testing. They isolate the impact of specific changes, ensuring that the observed differences are directly attributable to the tested element. This eliminates the influence of extraneous variables, leading to more reliable and accurate conclusions. A well-designed controlled experiment minimizes the risk of drawing erroneous conclusions from anecdotal evidence or subjective observations.
Different Types of A/B Tests
Various A/B tests can be conducted, each tailored to a specific business objective. The following table Artikels some common types:
Test Type | Description | Use Case |
---|---|---|
Button Color Test | Testing the impact of different button colors on click-through rates. | E-commerce websites, online marketing campaigns. |
Headline Test | Comparing different headlines to see which generates the most engagement. | Blog posts, landing pages, marketing emails. |
Layout Test | Assessing the effectiveness of different webpage layouts on user engagement. | E-commerce product pages, landing pages, blog pages. |
Image Test | Evaluating the performance of different images or visual elements in attracting user attention. | Social media posts, advertisements, marketing campaigns. |
Pricing Test | Comparing different pricing strategies to identify the most profitable approach. | E-commerce websites, subscription services, SaaS companies. |
Choosing the Right Metrics for A/B Testing
A/B testing is only as effective as the metrics you use to measure its success. Selecting the right key performance indicators (KPIs) is crucial for accurately assessing the impact of changes and drawing meaningful conclusions. Without appropriate metrics, you risk misinterpreting results and making decisions based on flawed data. This section will delve into the importance of choosing relevant KPIs, examining different types of tests, and understanding how to measure success effectively.Choosing the right metrics is vital to ensure that your A/B tests are measuring what truly matters.
If you track the wrong metrics, you could be optimizing for the wrong thing, ultimately hindering your overall goals. This meticulous process involves aligning your metrics with your specific objectives, ensuring a direct link between the changes you’re testing and the results you’re aiming for.
Significance of Relevant Metrics
Choosing the right metrics is paramount to the success of any A/B test. If the metrics don’t align with the test’s objectives, the results will be meaningless. For instance, if you’re testing a new call-to-action button, but your metric is website traffic, you might not accurately assess the button’s effectiveness on conversions. The goal is to measure the impact on the desired outcome, whether it’s increased sales, higher click-through rates, or improved user engagement.
Key Performance Indicators (KPIs) for Various A/B Tests
Different types of A/B tests require different KPIs. Understanding the appropriate metrics for each type is crucial. For example, a website test might focus on conversion rates, while an app test might concentrate on user retention rates. Marketing tests often target click-through rates, open rates, or other engagement metrics.
Measuring Success in an A/B Test
Success in an A/B test is defined by achieving statistically significant results that support a clear decision. This involves analyzing the data collected from the test, comparing the results between the different variations, and determining whether the observed differences are likely due to chance or a genuine effect. This analysis typically involves statistical tests to assess the significance of the results.
Common KPIs for A/B Tests
This table Artikels common KPIs for A/B tests, their descriptions, and formulas (where applicable).
KPI | Description | Formula (if applicable) |
---|---|---|
Conversion Rate | Percentage of visitors who complete a desired action (e.g., purchase, sign-up). | (Number of conversions / Number of visitors) – 100 |
Click-Through Rate (CTR) | Percentage of users who click on a specific link or advertisement. | (Number of clicks / Number of impressions) – 100 |
Bounce Rate | Percentage of visitors who leave a website after viewing only one page. | (Number of single-page visits / Total visits) – 100 |
Average Session Duration | Average time spent by a user on a website or app during a session. | Total session duration / Number of sessions |
User Engagement | Overall interaction with a website or app, encompassing various metrics. | Calculated based on metrics like page views, time on site, and click-through rates. |
Statistical Significance in Evaluating Results
Statistical significance is crucial in evaluating A/B test results. It determines whether the observed difference between variations is likely due to a real effect or just random chance. A statistically significant result indicates that the observed difference is unlikely to have occurred by chance.
“A p-value less than 0.05 is commonly used as a threshold for statistical significance, meaning there’s less than a 5% chance that the observed difference is due to random variation.”
Failing to account for statistical significance can lead to incorrect conclusions, potentially leading to wasted resources on ineffective changes.
Illustrative Examples of A/B Tests
A/B testing is a powerful tool for optimizing websites, apps, and marketing campaigns. By comparing different versions of a design or message, businesses can identify what resonates best with their target audience and drive better results. Understanding how these tests are applied in real-world scenarios is crucial for effectively leveraging this method.By analyzing the results of A/B tests, businesses can make data-driven decisions that improve user experience, increase conversion rates, and boost overall performance.
E-commerce Website A/B Tests
Understanding user behavior on e-commerce sites is paramount for driving sales. A/B tests allow you to precisely evaluate the impact of different design elements. For example, you can test various product layouts to determine which arrangement encourages more add-to-cart actions. Another common test involves contrasting different call-to-action (CTA) buttons. This could involve variations in color, text, or even button shape.
Test Goal | Variations Tested | Metrics Measured | Expected Results |
---|---|---|---|
Increase add-to-cart rate for product pages | Two different product layouts: One with a prominent “Add to Cart” button near the product image, the other with a “Buy Now” button below the product description. | Click-through rate to “Add to Cart” button, average order value, time spent on product page | The layout with the prominent “Add to Cart” button near the image may result in a higher add-to-cart rate. |
Boost conversion rates on product pages | Two different call-to-action (CTA) buttons: One using a vibrant red “Buy Now” button, the other a classic blue “Shop Now” button. | Conversion rate, cart abandonment rate, bounce rate | The button color that generates more conversions, or the one that keeps users on the page for a longer duration, will be considered the winner. |
Mobile App A/B Tests
Optimizing mobile app experiences is crucial for user engagement and retention. A/B tests help you find the perfect balance between functionality and user appeal. A/B tests can evaluate various button styles or notification frequency to determine what resonates best with the app’s target audience.
I’ve been diving deep into A/B testing lately, and I’m finding some amazing examples that are truly worth checking out. One particularly interesting development is the launch of Google’s new Swirl 3D ads, as highlighted in google swirl 3d ads ignite friday. While the new ad format is exciting, I’m keen to see how these translate into concrete A/B test results.
This got me thinking – what are the top 10 A/B testing examples that are truly impactful? Let’s explore some of those.
- Button Style Testing: Comparing different button colors, sizes, and shapes to see which leads to a higher click-through rate.
- Notification Frequency Testing: Examining how different notification schedules (e.g., daily vs. weekly) affect user engagement and app usage.
Marketing Campaign A/B Tests
A/B testing can significantly impact the effectiveness of marketing campaigns. Testing different ad copy and landing page designs can reveal the most engaging messages for your target audience.
- Ad Copy Testing: Analyzing different ad headlines, descriptions, and calls-to-action to determine which resonates most with your target audience.
- Landing Page Design Testing: Comparing variations in layout, imagery, and calls-to-action to see which landing page design converts more visitors into customers.
Implementing A/B Tests Effectively
A/B testing is a powerful tool for optimizing website design, marketing campaigns, and product features. However, simply setting up a test isn’t enough. Effective A/B testing requires careful planning, execution, and analysis to yield meaningful results. This section details the critical steps for implementing A/B tests successfully, from initial setup to data interpretation.Effective A/B testing hinges on meticulous planning and execution.
Thorough preparation minimizes the risk of errors and maximizes the likelihood of obtaining accurate and reliable results. It’s not just about choosing a variation; it’s about strategically designing the test to answer specific questions and measure the impact of those changes accurately.
Setting Up A/B Tests
Proper setup is fundamental to obtaining valid and reliable results from A/B tests. It involves defining clear objectives, creating variations, and strategically allocating traffic. Understanding the specific metrics that need to be tracked and defining the success criteria are crucial components.
- Defining Objectives: Clearly state the goals of the test. Are you aiming to increase conversion rates, boost click-through rates, or improve customer satisfaction? Specific and measurable objectives provide a framework for the entire testing process.
- Creating Variations: Develop distinct variations of the original design or element being tested. These variations should focus on specific changes, such as a different headline, call-to-action button, or layout. Focus on one change at a time for easier analysis.
- Traffic Allocation: Strategically allocate traffic between the variations. Randomization is key to ensuring that each variation receives a statistically significant number of users. Tools like Google Optimize can help with this.
- Metric Selection: Identify the key performance indicators (KPIs) that will be used to measure the success of each variation. Common metrics include conversion rates, click-through rates, bounce rates, and average session duration. Choose the metrics that directly align with your objectives.
Running A/B Tests
A smooth and well-executed test is essential for gaining valuable insights. A methodical approach to running A/B tests guarantees accurate data collection and analysis.
- Monitoring the Test: Continuously track the performance of each variation throughout the test duration. This enables early detection of significant performance differences. Tools such as Google Analytics provide real-time data insights.
- Data Collection: Ensure that all relevant data is collected and stored appropriately. The data should be accurately recorded and stored, and should align with the chosen metrics.
- Maintaining Traffic Allocation: Ensure that the predetermined traffic allocation remains consistent. This is crucial for accurate comparisons and prevents bias in the results.
Using Tools and Software for A/B Testing
Leveraging the right tools can significantly streamline the A/B testing process. These tools automate many of the steps, improving efficiency and accuracy.
- Google Optimize: A powerful tool for creating and managing A/B tests directly within a website. It provides detailed analytics and reporting, allowing for efficient tracking of test results.
- VWO (Visual Website Optimizer): Another popular option, providing advanced features for complex tests and analysis. It offers robust reporting and customizable dashboards.
Sample Size in A/B Testing
The sample size directly impacts the accuracy and reliability of the A/B test results. A smaller sample size can lead to unreliable conclusions, while a larger sample size increases the likelihood of obtaining statistically significant results. A critical analysis of the required sample size is necessary.
The appropriate sample size is crucial for statistical significance. A larger sample size generally yields more accurate results.
Analyzing A/B Test Results
Analyzing A/B test results is crucial for extracting actionable insights. This involves statistical analysis and interpreting the findings within the context of the test objectives.
- Statistical Significance: Determine whether the observed differences between variations are statistically significant. Tools can help determine the probability that the results occurred by chance. Statistical significance, usually measured by p-value, helps determine whether differences are real or due to random variation.
- Interpreting Findings: Analyze the results in the context of the original objectives. Was the desired outcome achieved by one of the variations? What specific elements of the variation were responsible for the positive or negative results? Was there any unexpected outcome? Carefully analyze the results to draw relevant conclusions.
Common Pitfalls and How to Avoid Them
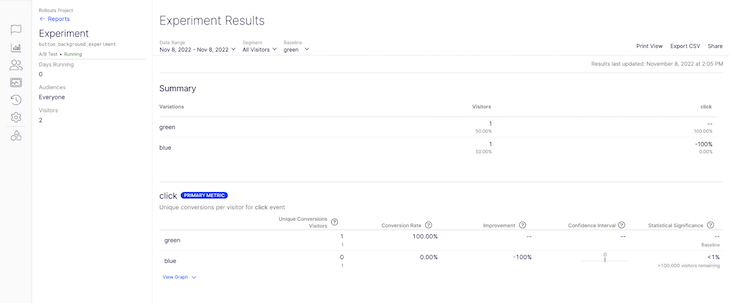
A/B testing, while powerful, is susceptible to various pitfalls. Understanding these potential errors and implementing preventative measures is crucial for obtaining accurate and reliable results. Ignoring these issues can lead to wasted resources, incorrect conclusions, and ultimately, poor business decisions. This section details common pitfalls and strategies to avoid them.Effective A/B testing relies on rigorous methodology. A clear understanding of potential biases and confounding variables is paramount to drawing valid inferences from the results.
Ever wanted to boost your conversion rates? Learning about the 10 best A/B test examples is a fantastic starting point. But, if you’re looking to expand your reach, checking out the latest on Twitter carousel ads might be key, as seen in this breaking news piece on breaking news how to promote your brand with twitter carousel ads.
Ultimately, understanding how these powerful tools work together can significantly improve your A/B testing strategies. So, dive into those 10 best A/B test examples – you won’t regret it!
This section will Artikel the key areas of concern and provide practical steps to mitigate risks.
Bias in A/B Testing, 10 best a b test examples you must try yourself
Bias in A/B testing arises when the test results are skewed in favor of one variation over another, due to factors outside the intended variable being tested. This can result in misleading conclusions about which variation performs better.
- Selection Bias: This occurs when the participants in the test are not representative of the target audience. For example, if the test is promoted to a specific demographic or location, the results might not be generalizable to the broader user base. Careful consideration of the target audience and representative sampling is crucial for avoiding selection bias.
- Confirmation Bias: Researchers might unconsciously favor the variation they believe will perform better, leading to an interpretation of the data that confirms their preconceived notions. Maintaining objectivity and using a structured approach to data analysis is essential for mitigating this bias.
- Observer Bias: This occurs when the researchers analyzing the data have preconceived notions about the outcomes, potentially influencing their interpretation of the results. Implementing a standardized data analysis protocol and using multiple researchers to review the data can help reduce observer bias.
Confounding Variables
Confounding variables are external factors that affect both the independent variable (the change being tested) and the dependent variable (the outcome being measured). If these variables are not accounted for, the results of the A/B test can be misleading.
- External Factors: External events, such as a marketing campaign or a change in website traffic patterns, can influence user behavior. Careful planning and execution of the test are crucial to isolate the effect of the tested variation.
- Seasonality: User behavior often varies depending on the time of year or specific holidays. A/B tests should ideally be run consistently across similar time periods to avoid misinterpreting seasonal effects as a result of the variation being tested.
- Changes in Platform or User Base: Updates to the website or changes in the user base can affect results. Consider the potential impact of such changes and adapt the testing methodology accordingly.
Poor Experimental Design
A poorly designed A/B test can lead to inaccurate or inconclusive results. The design must be meticulously planned to ensure that the test accurately measures the impact of the variation being tested.
So, you’re diving into A/B testing? Checking out 10 great examples is a fantastic start, but to truly understand their impact, you need to master conversion test tracking. Understanding how to effectively track conversions is key to analyzing the success of your A/B tests. This crucial aspect is often overlooked, but a tool like conversion test tracking helps you see which variations are truly driving more conversions.
This will help you fine-tune your strategies and get the most out of your A/B testing efforts. Ultimately, these 10 examples, combined with strong conversion test tracking, will elevate your results.
- Insufficient Sample Size: A small sample size can lead to statistically insignificant results. A larger sample size increases the likelihood of identifying a true difference between variations.
- Insufficient Duration: A/B tests need sufficient time to capture enough data to establish meaningful trends. An overly short test period may fail to capture long-term effects or patterns.
- Lack of Control Group: The control group serves as a baseline for comparison. Without a control group, it is impossible to determine whether the observed changes are due to the variation or other external factors.
Importance of the Control Group
The control group is crucial in A/B testing as it provides a baseline against which the performance of the variations can be compared. Without a control group, it is impossible to isolate the effect of the tested variation.
A well-defined control group allows for a fair assessment of the impact of the variation, minimizing the risk of misinterpreting the results.
A clear understanding of these pitfalls and proactive measures to avoid them are vital for achieving accurate and reliable results from A/B testing.
A/B Testing Best Practices: 10 Best A B Test Examples You Must Try Yourself
A/B testing, when done correctly, can provide invaluable insights into user behavior and optimize your website or application for maximum impact. However, simply running tests isn’t enough. Effective A/B testing demands a structured approach, adhering to best practices to ensure reliable and actionable results. This section details crucial steps and strategies for designing, executing, and interpreting A/B tests effectively.Implementing A/B testing requires meticulous planning and execution.
A well-defined strategy, focused on clear goals and measurable outcomes, is paramount to success. Failing to establish a clear direction can lead to inconclusive results and wasted resources.
Designing Effective Variations
Crafting compelling variations is critical to successful A/B testing. Simply changing colors or button text isn’t enough. Effective variations need to target specific user needs and preferences, driven by hypotheses based on prior data or user feedback. This involves understanding the user journey and identifying pain points to address.
- Focus on a Single Variable: Each variation should alter only one element at a time. This isolates the impact of the change, enabling accurate attribution of results.
- Consider User Experience: A/B testing shouldn’t sacrifice user experience. Variations should enhance, not disrupt, the user journey. Changes must be subtle and intuitive to encourage adoption.
- Test Compelling Hypotheses: Formulate hypotheses based on previous data or user feedback. Test variations that are likely to yield significant improvements. For example, if you suspect a change in call-to-action color will increase conversions, test that specific variation.
Defining Clear Goals and Hypotheses
Before launching any A/B test, establishing clear goals and hypotheses is crucial. This ensures that the test aligns with specific objectives and that results can be accurately interpreted. A well-defined hypothesis provides a framework for evaluating the success or failure of the variations.
- Establish Specific Goals: Identify measurable goals for the A/B test. For instance, increase conversion rates, improve time-on-site, or reduce bounce rates. These goals must be quantifiable.
- Formulate Testable Hypotheses: Create testable hypotheses about the effect of the variations on the chosen metrics. For example, “Changing the button color from blue to green will increase conversion rates by 10%.”
- Prioritize Relevant Metrics: Choose the correct metrics to track. For example, if the goal is to improve conversion rates, metrics like conversion rate, click-through rate, and time spent on the page should be monitored. Focus on metrics that are directly tied to the business objectives.
Interpreting and Acting Upon Results
Interpreting the results of an A/B test is a critical step. Carefully analyze the data and ensure that the conclusions drawn are statistically sound. Avoid drawing conclusions based on small sample sizes or insignificant results.
- Statistical Significance: Use statistical methods to determine if the observed differences between variations are statistically significant. Don’t rely on small differences; ensure the variations are significant enough to be reliable.
- Confidence Intervals: Understand confidence intervals to assess the uncertainty in the results. A wider confidence interval indicates a higher level of uncertainty in the observed differences.
- Actionable Insights: Based on the statistically significant results, determine whether to implement the winning variation. Consider the potential impact on the business and user experience.
Maintaining Rigor and Control
Maintaining rigor and control throughout the A/B testing process is crucial for reliable results. Careful monitoring, proper randomization, and adherence to best practices ensure the validity of the conclusions.
- Randomization: Ensure that users are randomly assigned to either the control or variation groups to prevent bias.
- Control Group: A control group provides a baseline for comparison to evaluate the effectiveness of the variation.
- Monitoring and Adjustment: Continuously monitor the test results and be prepared to adjust the test parameters or stop the test if necessary.
Case Studies
A/B testing isn’t just a theoretical concept; it’s a powerful tool used by countless companies to drive real-world improvements. Understanding how these companies have implemented A/B tests, the results they achieved, and the strategies they employed provides valuable insights for anyone looking to leverage this technique effectively. These case studies offer practical examples of A/B testing success, showcasing the impact it can have on various aspects of a business.Real-world A/B testing success stories demonstrate how companies have optimized their operations, boosted user engagement, and ultimately increased revenue by meticulously evaluating different approaches.
Examining the strategies used, the metrics tracked, and the results achieved allows us to learn from these successful implementations and apply similar principles to our own A/B testing initiatives.
Examples of Successful A/B Tests
Numerous companies have successfully utilized A/B testing to enhance their marketing campaigns, improve website design, and optimize user experiences. These examples highlight the versatility and impact of this powerful technique.
- Amazon: Amazon famously utilizes A/B testing to optimize product placement, recommendation algorithms, and checkout processes. By meticulously evaluating various design elements and presenting different product sets, Amazon has fine-tuned the user experience, leading to significant increases in conversion rates and customer satisfaction. Their approach is characterized by a large-scale, data-driven methodology that tracks every detail. They are known to continuously refine their testing procedures to improve the effectiveness of their A/B testing process.
This constant refinement ensures that the testing process remains relevant and applicable to the ever-changing landscape of online shopping.
- Netflix: Netflix employs A/B testing to personalize recommendations, tailor user interfaces, and optimize content suggestions. They analyze user behavior and preferences to create different versions of their platform, enabling them to determine which variations are most effective in attracting and retaining subscribers. The platform is updated and improved based on the feedback from these A/B tests, resulting in a more intuitive and engaging user experience.
Netflix’s testing approach emphasizes personalization and caters to the ever-evolving preferences of their vast user base.
- Airbnb: Airbnb utilizes A/B testing to optimize its search algorithms, pricing strategies, and communication channels. They test different versions of their search results pages, adjust pricing models based on demand, and experiment with different communication methods to understand what resonates best with potential hosts and guests. Their results often demonstrate a strong correlation between the A/B tests’ success and increased bookings and user satisfaction.
Comparative Analysis of A/B Testing Strategies
A variety of strategies are employed by companies during A/B testing. A comparison of these approaches provides valuable insights into the different methodologies that companies use.
Company | A/B Testing Strategy | Key Metrics | Results |
---|---|---|---|
Amazon | Large-scale, data-driven, continuous improvement | Conversion rate, customer satisfaction, revenue | Significant increases in conversion rates, improved customer satisfaction, and substantial revenue growth |
Netflix | Personalized recommendations, tailored UI, optimized content suggestions | User engagement, subscriber retention, viewing time | Increased user engagement, improved subscriber retention, and enhanced viewing experience |
Airbnb | Optimized search algorithms, pricing strategies, communication channels | Booking rates, user satisfaction, host engagement | Increased booking rates, improved user satisfaction, and strengthened host engagement |
Impact on Business Outcomes
Successful A/B testing initiatives yield tangible results that positively impact various business outcomes. Companies often see improvements in key metrics like conversion rates, customer satisfaction, and revenue. The specific impact will vary depending on the industry, the specific tests performed, and the company’s overall strategy.
Wrap-Up
In conclusion, mastering A/B testing is crucial for any business looking to optimize performance and drive growth. By understanding the principles, implementing the best practices, and learning from real-world examples, you can confidently run impactful tests that yield tangible results. The 10 examples highlighted in this guide are just the starting point; the key is to adapt and iterate, continually refining your strategies based on data-driven insights.