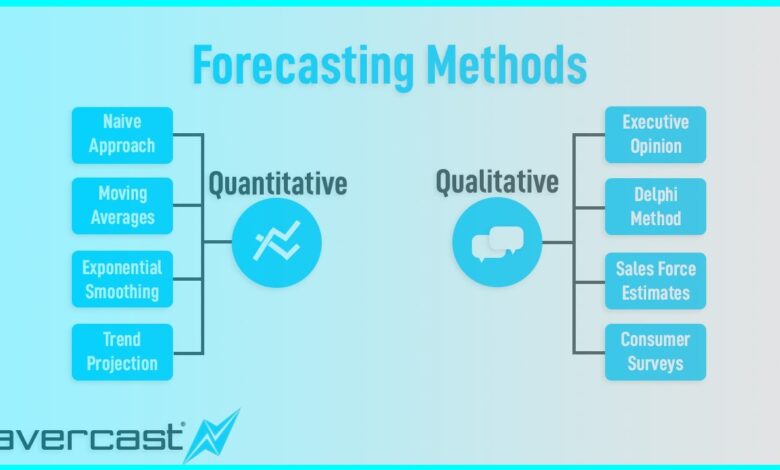
The Forecaster Method A Comprehensive Guide
The forecaster method offers a powerful approach to predicting future trends and outcomes. This method, used across diverse industries, provides a structured framework for forecasting, moving beyond simple guesswork. It delves into historical data, analyzes current trends, and identifies potential future challenges, ultimately helping you anticipate and prepare for what’s to come. This guide explores the core principles, historical context, and practical applications of the forecaster method.
We’ll dissect the components and procedures involved, examining its strengths and weaknesses compared to other forecasting techniques. Understanding data requirements and implementation strategies is crucial for successful use. We’ll also illustrate the method with real-world examples, demonstrating its practical application in diverse fields.
Defining the Forecaster Method
The forecaster method is a structured approach to predicting future outcomes. It encompasses a wide range of techniques, from simple trend analysis to complex econometric models. Crucially, it goes beyond mere guesswork, relying on data analysis, statistical modeling, and expert judgment to provide reasoned estimations. This method is distinct from simply expressing opinions; it’s a disciplined process designed to inform decisions based on probabilities.This method is valuable in a variety of fields, from business forecasting to scientific research.
By understanding the underlying principles and diverse techniques within the forecaster method, one can effectively utilize this framework to anticipate and adapt to future conditions.
Key Characteristics of the Forecaster Method
The forecaster method distinguishes itself from other forecasting approaches through its emphasis on systematic data analysis, model building, and validation. It’s not simply about looking at past trends; it’s about identifying patterns, understanding underlying drivers, and using those insights to project future outcomes. A key element is the explicit consideration of uncertainties and potential risks associated with predictions.
This methodical approach enhances the accuracy and reliability of forecasts.
Types of Forecaster Methods, The forecaster method
Different forecaster methods employ various techniques to achieve predictions. This table Artikels some common types, highlighting their characteristics, examples, and strengths.
Method Type | Description | Example | Strengths |
---|---|---|---|
Trend Analysis | Identifies patterns in historical data to project future trends. It assumes that past trends will continue into the future. | Predicting future sales based on historical sales figures, assuming consistent growth or decline. | Simple to understand and implement, requiring minimal data. |
Econometric Modeling | Uses statistical methods to model the relationships between various economic variables. These models can be complex, incorporating multiple variables and relationships. | Predicting inflation based on factors like interest rates, unemployment, and consumer spending. | Provides a more nuanced understanding of the underlying drivers of a phenomenon, offering greater accuracy if the relationships are well-understood. |
Time Series Analysis | Focuses on identifying patterns and seasonality in time-dependent data. It’s particularly useful for forecasting cyclical events. | Predicting monthly electricity demand based on past consumption patterns and seasonal variations. | Effective for data with inherent time-dependent patterns and seasonality. |
Delphi Method | A forecasting technique that involves collecting and aggregating expert opinions on a given topic. | Forecasting the impact of a new technology on a market, gathering input from leading experts in the field. | Leverages the collective wisdom of experts, often providing a broader range of perspectives than traditional methods. |
Historical Context and Evolution
The forecaster method, a cornerstone of many fields from finance to meteorology, isn’t a static entity. Its development mirrors the evolution of computational power, data availability, and the very understanding of the systems being forecast. This journey, from rudimentary estimations to sophisticated algorithms, reveals a fascinating interplay between theory and practice.The method has been continuously refined and adapted to suit diverse needs, from predicting crop yields to anticipating market trends.
This evolution is marked by a series of crucial milestones and advancements that significantly impacted the accuracy and efficiency of forecasting.
Early Forms of Forecasting
Early forms of forecasting were largely intuitive and qualitative. Individuals relied on experience, observation, and rudimentary data analysis to make predictions. For example, ancient civilizations observed celestial patterns to predict agricultural cycles, and merchants assessed market conditions based on historical trends. These methods, while often inaccurate, laid the foundation for more systematic approaches.
The Rise of Quantitative Methods
The 20th century witnessed a significant shift towards quantitative methods. The development of statistical tools and techniques like regression analysis and time series analysis provided a more structured framework for forecasting. These methods allowed for the quantification of relationships between variables, leading to more precise predictions. Early examples include the use of moving averages to predict demand in manufacturing.
Advancements in Computational Power
The advent of computers dramatically accelerated the development of forecasting methods. Complex algorithms and sophisticated models, previously impractical, became feasible. This allowed for the incorporation of vast amounts of data and the exploration of intricate relationships, leading to more accurate and comprehensive forecasts.
Evolution Across Industries
The forecaster method has evolved alongside the needs of different industries. In finance, the development of econometric models helped predict market fluctuations. In meteorology, the introduction of sophisticated weather models significantly improved the accuracy of weather forecasts. Similarly, in agriculture, the use of crop models and soil analysis techniques revolutionized yield predictions.
A Timeline of Key Events
Year | Event | Impact |
---|---|---|
1800s | Initial development of statistical methods | Foundation for quantitative forecasting |
1900s | Introduction of time series analysis | More structured approach to forecasting |
Mid-20th Century | Development of computer models | Enabled complex calculations and incorporation of large datasets |
1970s-Present | Refinement of machine learning algorithms | Improved accuracy and adaptability of forecasts in various fields |
Applications and Use Cases
The forecaster method, with its ability to analyze historical data and project future trends, finds widespread applications across various industries. Its adaptability and versatility make it a valuable tool for informed decision-making in diverse contexts. From predicting market fluctuations to forecasting scientific phenomena, the method offers a structured approach to anticipate outcomes and prepare for potential challenges.The forecaster method’s strength lies in its ability to synthesize complex information and distill it into actionable insights.
By identifying patterns and trends, it allows individuals and organizations to make proactive adjustments, optimize resource allocation, and mitigate risks. This approach is especially crucial in fields where accurate predictions are essential for success, such as business, finance, and scientific research.
The forecaster method, while powerful, often requires a deep understanding of market trends. To truly maximize its potential, a skilled SEO Project Manager like the ones wanted at Ignite Visibility ( seo project manager wanted join ignite visibility ) is crucial. They can refine forecasts by analyzing data, identifying crucial market shifts, and tailoring strategies for optimal results.
Ultimately, this approach leads to more accurate and effective forecasting.
Forecasting in Business
Businesses rely heavily on forecasting to anticipate demand, manage inventory, and make strategic decisions. Sales forecasts, for instance, help companies determine production levels and staffing needs. By anticipating future sales trends, companies can optimize their operations and avoid costly overstocking or understocking scenarios. A company predicting high demand for a product can adjust production accordingly, ensuring timely delivery and maximizing revenue.
Likewise, inventory forecasting helps manage storage costs and prevent stockouts. Accurate forecasting can also influence pricing strategies and marketing campaigns, leading to increased profitability. A well-defined forecasting process provides a crucial framework for strategic planning, ensuring that business decisions are grounded in realistic projections.
Forecasting in Finance
Financial forecasting plays a critical role in investment strategies, risk management, and financial planning. Accurate forecasts of economic indicators, such as inflation or interest rates, enable investors to make informed decisions about portfolio diversification and asset allocation. By anticipating market fluctuations, financial institutions can assess and manage potential risks effectively. Moreover, accurate financial forecasts are instrumental in budgeting and financial planning for individuals and organizations.
Forecasting allows for realistic financial projections, helping to allocate resources effectively and avoid financial distress.
Forecasting in Science
The forecaster method is not limited to the business and finance sectors. In scientific research, it enables researchers to predict phenomena, test hypotheses, and understand complex systems. For example, climate scientists use forecasting models to project future climate change scenarios and their potential impacts. These models, incorporating vast datasets of historical weather patterns, can provide insights into future temperature changes, precipitation patterns, and sea-level rise.
Similar forecasting techniques are used in various scientific disciplines to understand and predict natural events and biological processes.
Use Case Categorization by Industry
Industry | Use Case | Description | Impact |
---|---|---|---|
Retail | Sales Forecasting | Predicting future sales of products based on historical data and market trends. | Optimized inventory management, efficient resource allocation, and increased profitability. |
Manufacturing | Demand Forecasting | Anticipating future demand for products to optimize production schedules and resource allocation. | Reduced costs associated with overproduction or underproduction, improved supply chain efficiency, and increased customer satisfaction. |
Agriculture | Crop Yield Forecasting | Estimating future crop yields based on weather patterns, soil conditions, and historical data. | Improved planning for planting, harvesting, and resource allocation, minimizing risks associated with natural disasters, and ensuring food security. |
Energy | Electricity Demand Forecasting | Predicting future electricity demand to optimize energy production and distribution. | Efficient grid management, reduced energy waste, and improved public safety. |
Components and Procedures
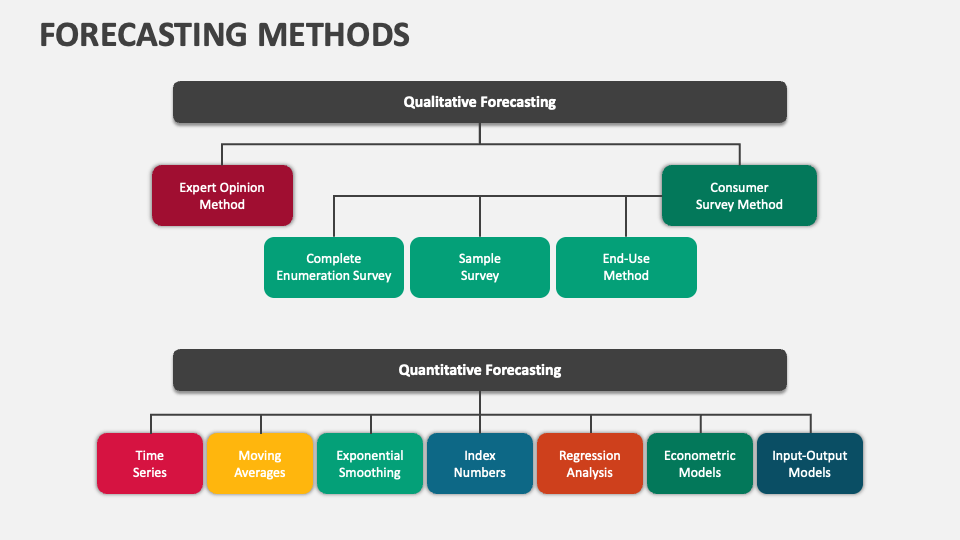
The forecaster method, a powerful tool for predicting future trends, relies on a structured approach. Understanding its components and procedures is crucial for effective application and achieving accurate results. This section delves into the essential elements of the method, outlining the steps involved and the factors influencing its accuracy.The forecaster method is not a one-size-fits-all solution. Its application varies based on the specific industry, the nature of the data being analyzed, and the desired level of accuracy.
However, common components and a structured procedure can enhance its effectiveness.
Essential Components
The forecaster method typically involves several key components. These components provide a framework for data collection, analysis, and prediction. Data quality and accuracy are paramount to the success of the entire process.
- Data Collection: This involves gathering relevant historical data, including market trends, sales figures, economic indicators, and other pertinent information. The comprehensiveness and reliability of the data directly impact the accuracy of the predictions.
- Data Analysis: This step focuses on examining the collected data to identify patterns, trends, and relationships. Statistical methods, such as regression analysis or time series analysis, are often employed to model the data and extract meaningful insights.
- Model Building: Based on the analysis, a predictive model is created. This model encapsulates the relationships discovered in the data, enabling estimations of future outcomes. Different models, such as linear regression, exponential smoothing, or ARIMA, may be suitable depending on the characteristics of the data.
- Validation and Refinement: The model’s accuracy is assessed through validation techniques. This step involves comparing the model’s predictions with actual outcomes to identify any discrepancies and refine the model to improve its predictive capabilities. Adjustments are made to enhance the model’s accuracy and minimize errors.
- Prediction and Interpretation: The validated model is used to generate forecasts for future periods. These predictions are then interpreted to gain actionable insights and support strategic decision-making.
Step-by-Step Procedures
A typical application of the forecaster method follows a systematic procedure.
- Define the Objective: Clearly identify the purpose of the forecast. What specific aspect of the future are you trying to predict? Examples include predicting sales volume, estimating market share, or anticipating demand for a product.
- Data Collection and Preparation: Gather relevant historical data. Ensure data accuracy and completeness. Prepare the data by cleaning, transforming, and organizing it into a suitable format for analysis.
- Model Selection and Training: Choose a suitable forecasting model based on the characteristics of the data and the desired level of accuracy. Train the model using the prepared data.
- Model Validation: Assess the model’s performance using validation techniques. Evaluate the model’s accuracy by comparing its predictions with actual outcomes.
- Refinement and Iteration: Refine the model based on the validation results. Iterate on the model and its parameters to enhance accuracy and reliability. Consider external factors and adjust accordingly.
- Prediction and Communication: Use the refined model to generate forecasts for the desired future period. Communicate the forecast results clearly and concisely to stakeholders.
Factors Affecting Accuracy
Numerous factors can influence the accuracy of the forecaster method.
- Data Quality: Inaccurate or incomplete data can significantly compromise the accuracy of the predictions. Errors, omissions, or inconsistencies in the data will propagate to the forecasts.
- Model Selection: Choosing an inappropriate model for the specific data can lead to inaccurate predictions. The model should align with the nature of the data and the desired level of accuracy.
- External Factors: Unforeseen events, economic shifts, or changes in consumer behavior can disrupt the patterns identified in the historical data. These factors need to be considered and accounted for in the model.
- Model Assumptions: Forecasting models often rely on assumptions. Violations of these assumptions can lead to inaccurate forecasts.
- Forecast Horizon: Longer forecast horizons are typically associated with lower accuracy. The further into the future the forecast extends, the more prone it is to error.
Illustrative Flowchart
A flowchart can visually represent the procedure:
[Insert a simplified flowchart here illustrating the steps described above. Describe the flowchart’s components. For example: The flowchart begins with “Define Objective” at the top left and branches to “Data Collection” and “Data Preparation.” Each step is represented by a box, and arrows indicate the flow of the procedure.]
Strengths and Weaknesses
The forecaster method, while offering valuable insights, isn’t without its limitations. Understanding its strengths and weaknesses, and comparing them to other forecasting techniques, is crucial for effective application and informed decision-making. This section delves into the advantages and disadvantages of the forecaster method, providing a balanced perspective for potential users.
Key Strengths of the Forecaster Method
The forecaster method’s strength lies in its ability to leverage expert knowledge and subjective judgment. This approach allows for incorporating nuanced factors, like market trends, competitor actions, and technological advancements, that often aren’t captured by purely quantitative methods. Forecasting relies on human intuition and experience, which can be especially valuable when dealing with complex or novel situations. Furthermore, the method is adaptable and can be tailored to specific contexts, making it suitable for diverse applications.
This flexibility allows for a deeper understanding of the factors influencing the forecast.
Limitations of the Forecaster Method
Despite its strengths, the forecaster method has limitations. Subjectivity is a significant drawback, as individual biases can influence the forecast. Lack of standardized procedures and consistent methodologies across different forecasters can lead to inconsistent results. Furthermore, the forecaster method may struggle with large datasets or situations demanding extensive statistical analysis. The lack of quantifiable metrics can make it challenging to validate the forecast’s accuracy and reliability.
Comparison with Other Forecasting Techniques
Different forecasting techniques offer various strengths and weaknesses. For instance, quantitative methods, like time series analysis, excel at identifying patterns in historical data, but may struggle to account for unforeseen events. Expert judgment methods, while insightful, can suffer from bias and lack of consistency. A hybrid approach, combining elements of quantitative and qualitative methods, often yields more robust and accurate forecasts.
Comparison Table of Forecasting Methods
Method | Strengths | Weaknesses |
---|---|---|
Forecaster Method | Leverages expert knowledge, adaptable to specific contexts, considers nuanced factors, good for complex situations | Subjectivity can lead to bias, lack of standardized procedures, challenging to validate accuracy, may struggle with large datasets |
Time Series Analysis | Identifies patterns in historical data, quantifiable, relatively objective | May not account for unforeseen events, relies heavily on historical data, can be complex to implement |
Delphi Method | Reduces bias through anonymous feedback from experts, encourages diverse perspectives | Can be time-consuming, requires careful design, may not reflect individual expert confidence |
Regression Analysis | Identifies relationships between variables, quantifiable, allows for prediction | Assumes linear relationships, requires accurate data, can be affected by outliers |
Data Requirements and Considerations: The Forecaster Method
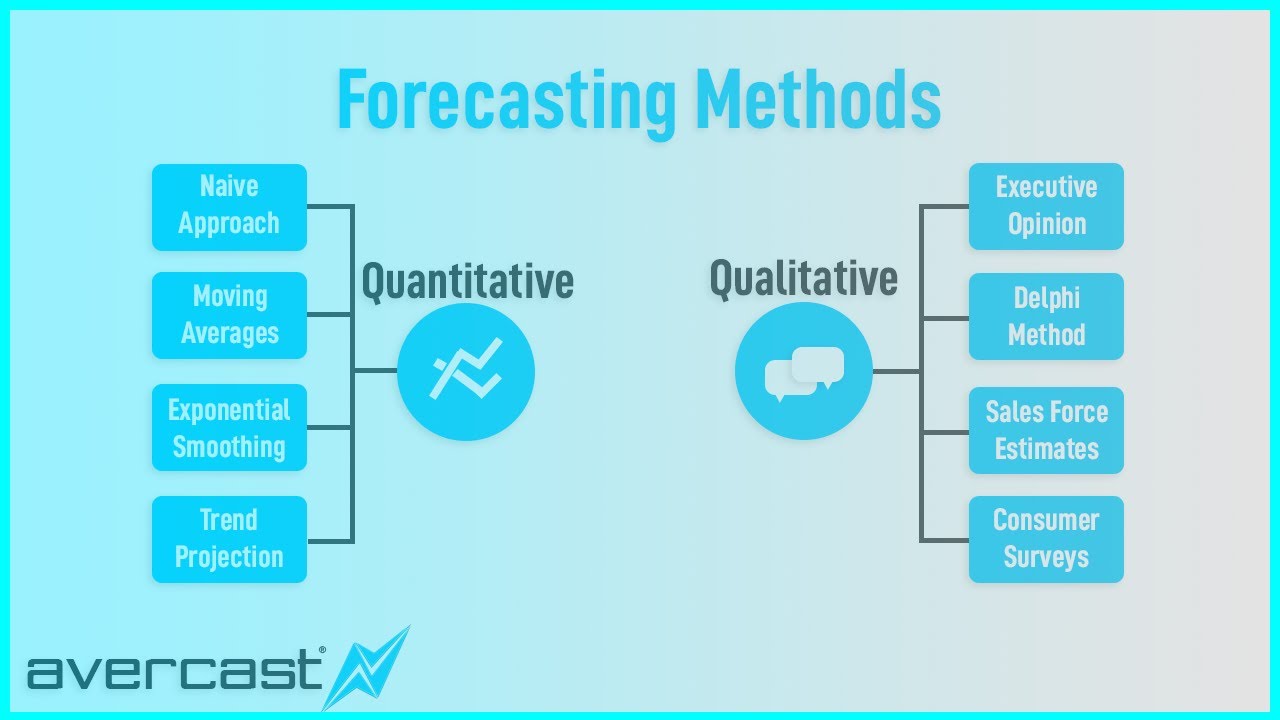
The forecaster method, at its core, relies heavily on the quality and accuracy of the data it processes. Without reliable input, the forecasts generated will be unreliable, potentially leading to poor decision-making. This section delves into the critical data requirements and the pitfalls associated with inadequate data, emphasizing the importance of data quality for successful forecasting.Effective forecasting hinges on appropriate data selection and handling.
Understanding the nature of the data and its potential limitations is crucial for building robust forecasting models. This section highlights the types of data needed, the significance of data quality, and strategies for managing potential issues arising from missing or inconsistent data formats.
Types of Data Required
Forecasting models often require various data types to generate accurate predictions. These include historical data, external factors, and potentially, specific data points related to the phenomenon being predicted. For instance, in predicting sales, historical sales figures, marketing campaign data, economic indicators, and seasonal trends would be necessary. Understanding the specific data needed is directly linked to the type of forecasting being conducted and the desired outcome.
Importance of Data Quality and Accuracy
Data quality is paramount in the forecaster method. Inaccurate or incomplete data will lead to erroneous forecasts. For example, if historical sales data contains errors or inconsistencies, the forecasting model will produce unreliable predictions. Ensuring data accuracy involves rigorous data validation, cleaning, and standardization processes.
Handling Missing Data
Missing data is a common issue in datasets. Different strategies can address this challenge, including imputation techniques, which estimate missing values based on existing data. For example, linear interpolation can be used to estimate missing values based on the trend of the surrounding data points. If a significant portion of the data is missing, the forecaster might need to consider alternative forecasting methods or adjust the scope of the analysis.
Handling Inconsistent Data Formats
Data inconsistency, such as differing units of measurement or inconsistent data entry formats, can significantly affect forecasting accuracy. For example, if sales figures are recorded in different currencies or using inconsistent date formats, the data needs to be standardized before it can be used in the forecasting model. A robust data preprocessing step is essential to transform the data into a usable format for the forecasting model.
Examples of Data Format Handling
Consider a dataset containing sales figures from different regions. If the data is in different currencies, it must be converted to a single currency before analysis. Similarly, if the dates are recorded in various formats (e.g., MM/DD/YYYY, DD/MM/YYYY), they need to be standardized to a consistent format. Tools like spreadsheets and specialized data management software can be used to manage and transform the data into the required format.
For instance, using a spreadsheet software’s built-in functions, the user can convert currencies and standardize dates, ensuring data integrity.
Implementation Strategies
Putting the forecaster method into practice requires careful planning and execution. A successful implementation hinges on understanding the unique needs of your organization and tailoring the method to fit existing workflows. This section details best practices, integration approaches, and crucial considerations before and during implementation.Implementing the forecaster method effectively involves more than just choosing a software package. It requires a fundamental shift in mindset, fostering a culture of data-driven decision-making and empowering personnel to leverage the method’s capabilities.
Best Practices for Implementation
Successful implementation relies on a number of best practices. These practices, when followed, maximize the method’s potential and minimize potential pitfalls.
The forecaster method, while useful for predicting trends, can sometimes fall short in pinpointing the precise nuances needed for success. That’s where search engine optimization specialists come in. They leverage intricate data analysis and tailored strategies to ensure websites rank higher in search results, ultimately driving targeted traffic. This refined approach, bolstered by expert knowledge, complements the forecaster method by ensuring accurate projections translate into real-world results.
- Establish Clear Objectives and KPIs: Defining specific, measurable, achievable, relevant, and time-bound (SMART) objectives is crucial. Key Performance Indicators (KPIs) should be clearly defined to track progress and measure the success of the implementation. This ensures the method directly addresses organizational needs and provides concrete metrics for evaluating performance.
- Develop a Comprehensive Training Program: Thorough training is essential for all personnel involved. Training should cover the theoretical underpinnings of the method, practical application, and the use of any associated software or tools. Training should also address potential pitfalls and common challenges. This empowers staff to confidently utilize the method and troubleshoot issues effectively.
- Pilot the Method in a Controlled Environment: A pilot project allows for testing the method in a controlled setting before full implementation. This helps identify potential issues, refine procedures, and adjust the method to best suit the specific context. This crucial step allows for adjustments and modifications before a broader rollout.
- Foster Data Culture and Collaboration: The forecaster method depends heavily on quality data. Creating a data-driven culture that prioritizes data accuracy and consistency is essential. Encourage open communication and collaboration between departments to ensure data sharing and alignment. This includes defining clear data ownership and responsibility to guarantee data quality.
Approaches for System Integration
Successful implementation often requires integrating the forecaster method into existing systems. A variety of approaches are available, each with its own advantages and considerations.
- API Integration: Leveraging Application Programming Interfaces (APIs) allows for seamless data exchange between the forecaster method system and existing databases or applications. This minimizes manual data entry and ensures data consistency. This approach is especially useful for large organizations with intricate data systems.
- Custom Scripting: In some cases, custom scripting can be employed to tailor the forecaster method to the specific requirements of an organization. This approach offers a high degree of customization, but also requires significant technical expertise. This is best suited for organizations with internal IT resources or dedicated programming staff.
- Data Warehousing: Creating a centralized data warehouse provides a unified source of data for the forecaster method. This ensures consistent data quality and accessibility across different departments. This approach enhances the efficiency of data analysis and reporting.
Implementation Checklist
This checklist helps to ensure a smooth and successful implementation.
The forecaster method, while powerful, often relies on robust data. Understanding how to structure that data for search engines is key, and that’s where need know schema org comes in handy. Knowing how to implement schema markup correctly will significantly improve the accuracy of forecasts, as it helps search engines better understand and interpret the information.
This ultimately leads to more accurate and reliable forecasts.
- Data Validation and Cleaning: Verify data accuracy and consistency, addressing any inconsistencies or missing values. This is a crucial step to ensure the quality of input data and the reliability of the forecasts.
- System Compatibility Assessment: Ensure compatibility with existing systems, software, and hardware. This includes evaluating data formats, security protocols, and other relevant factors. This avoids potential disruptions or compatibility issues.
- User Acceptance Testing (UAT): Conduct UAT with representative users to gather feedback on the usability and effectiveness of the system. This helps identify any issues or areas for improvement before full implementation.
- Security Protocols and Compliance: Implement robust security measures to protect sensitive data. This includes adhering to relevant data privacy regulations and industry standards.
Detailed Guide to System Setup
This guide provides a step-by-step approach to setting up a forecaster method system.
Step | Action |
---|---|
1 | Define Project Scope and Objectives: Clearly Artikel the project’s goals, target audience, and expected outcomes. |
2 | Data Collection and Preparation: Gather relevant historical data, ensuring its accuracy and consistency. |
3 | Model Selection and Parameterization: Choose an appropriate forecaster method and configure its parameters based on the collected data. |
4 | System Integration: Integrate the forecaster method system with existing data sources and applications. |
5 | Testing and Validation: Conduct thorough testing and validation to ensure accuracy and reliability. |
6 | Training and Documentation: Provide comprehensive training to relevant personnel and develop detailed documentation. |
7 | Deployment and Monitoring: Deploy the system and establish a monitoring process to track performance and make adjustments as needed. |
Illustrative Examples
The Forecaster Method, in its various forms, is not just a theoretical framework. Its practical application can be seen in numerous real-world scenarios, from predicting market trends to optimizing resource allocation. Understanding these real-world examples provides valuable insight into the method’s power and limitations.
A Real-World Example: Forecasting Retail Sales
Retailers consistently face the challenge of accurately predicting sales volume. The Forecaster Method, applied to this context, involves several key steps. First, historical sales data is meticulously examined, identifying trends and seasonality. This data might include weekly or monthly sales figures, broken down by product category and store location. Then, external factors like economic indicators, competitor activity, and marketing campaigns are incorporated into the forecasting model.
For instance, if a retailer anticipates a significant advertising campaign, this might influence the predicted sales volume. Finally, the model utilizes statistical techniques, such as regression analysis, to project future sales based on the identified patterns and external factors. The output is a detailed sales forecast for the upcoming period, including specific sales projections for each product category and store location.
Case Study: Successful Application in a Manufacturing Setting
A manufacturing company specializing in automotive parts experienced significant fluctuations in demand. The company employed the Forecaster Method to mitigate these fluctuations and improve production planning. The initial phase involved collecting and analyzing historical data on production, sales, and raw material costs. This involved data on daily production output, monthly sales figures, and the cost of raw materials.
The data was further analyzed to identify correlations between demand patterns and external factors like seasonal variations and economic indicators. For example, the company found that demand for certain parts was consistently higher during the summer months due to increased vehicle production. This insight allowed the company to adjust production schedules accordingly. The Forecaster Method helped the company to proactively adjust its production schedule to meet fluctuating demand, reducing inventory costs and minimizing stockouts.
The method also helped optimize raw material purchasing, leading to significant cost savings. The result was a more efficient and cost-effective production process, and a more stable supply chain.
Conclusion
In conclusion, the forecaster method provides a valuable tool for anyone needing to anticipate future outcomes. Its multifaceted approach, drawing on historical data, current trends, and potential challenges, equips users with a structured methodology to make informed decisions. By understanding the method’s components, procedures, and potential limitations, you can effectively utilize this powerful technique to achieve a more accurate and insightful forecast.
This guide provides a comprehensive overview, empowering you to confidently navigate the future with greater foresight.