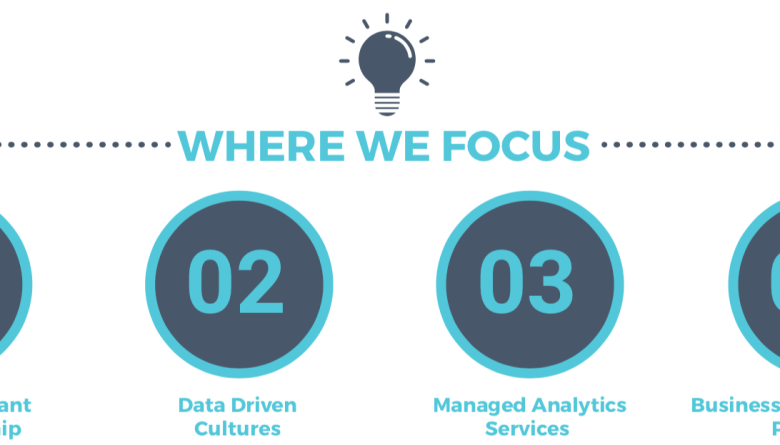
Analytics Audits and Data Integrity A Deep Dive
Analytics audits and data integrity are crucial for any organization relying on data-driven decisions. This deep dive explores the multifaceted nature of these audits, examining everything from defining the audits themselves to the crucial role of data integrity and the essential tools used. We’ll cover various aspects, including data collection, storage, transformation, and analysis, providing practical insights and actionable strategies to ensure the accuracy and reliability of your data.
A well-executed analytics audit not only validates the accuracy of your data but also helps identify vulnerabilities, optimize processes, and ultimately improve the trustworthiness of your analytical insights. This process ensures your data is reliable, allowing you to make sound business decisions and achieve your goals.
Defining Analytics Audits
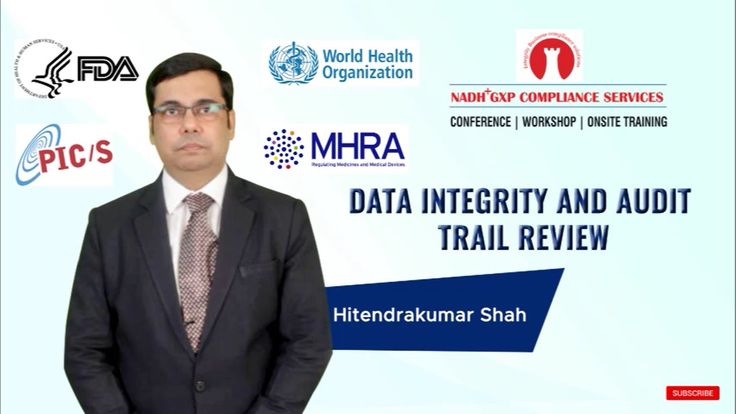
Analytics audits are systematic reviews of an organization’s analytics processes, data, and systems. They aim to ensure the accuracy, reliability, and effectiveness of the analytics function, which is crucial for informed decision-making. These audits are critical for identifying potential weaknesses, errors, or biases in the analytics pipeline and for establishing robust processes to maintain data integrity.A thorough analytics audit goes beyond simply checking for errors.
It investigates the entire lifecycle of data, from its collection and storage to its analysis and reporting. This holistic approach ensures that the insights derived from data are trustworthy and actionable. Furthermore, the audit helps establish a culture of data quality and integrity, benefiting the entire organization.
Types of Analytics Audits, Analytics audits and data integrity
Analytics audits encompass various types, each focusing on a specific aspect of the analytics process. Understanding these different types is crucial for tailoring the audit approach to the organization’s specific needs.
- Data Quality Audits: These audits assess the accuracy, completeness, consistency, and timeliness of the data used in analytics. They identify and address data issues like missing values, inconsistencies in formats, and inaccurate data entry. Data quality audits are essential to ensure that the insights derived from the data are reliable and meaningful. For example, a data quality audit might reveal that customer demographics are inconsistent across different data sources, leading to inaccurate segmentation models.
- Process Audits: These audits focus on the procedures and methodologies used in the analytics process. They evaluate the effectiveness of data collection, processing, analysis, and reporting methods. Process audits examine whether the steps in the analytical workflow are well-defined, documented, and consistently followed. For example, a process audit might reveal that the process for validating statistical models lacks documentation, leading to inconsistent results and an inability to track the source of the model’s errors.
- System Audits: These audits evaluate the technical infrastructure supporting the analytics function. They assess the security, performance, and reliability of the data storage, processing, and reporting systems. System audits ensure that the systems used for data storage, processing, and analysis are secure, scalable, and reliable. A system audit might reveal that the current data warehouse is not optimized for handling large volumes of data, leading to slow query response times.
Establishing Audit Criteria and Procedures
Defining clear criteria and procedures is paramount for the success of an analytics audit. This includes establishing specific metrics for evaluating data quality, defining the scope of the audit, and outlining the steps for each audit type. Without well-defined criteria and procedures, audits may lack focus and consistency, potentially missing critical issues.
Audit Type | Objectives | Scope | Key Procedures |
---|---|---|---|
Data Quality Audit | Ensure data accuracy, completeness, consistency, and timeliness. | Data sources, data fields, data transformations. | Data profiling, data validation, data cleansing. |
Process Audit | Evaluate the effectiveness of analytical methodologies. | Data collection, processing, analysis, reporting. | Process mapping, workflow review, documentation analysis. |
System Audit | Assess the reliability and security of analytics systems. | Data storage, processing, reporting systems, infrastructure. | System performance testing, security assessments, access controls review. |
Data Integrity in Audits: Analytics Audits And Data Integrity
Data integrity is the bedrock of any reliable analytics audit. Without accurate and trustworthy data, the entire audit process becomes suspect, leading to flawed conclusions and potentially costly errors in decision-making. This critical aspect of auditing ensures that the insights gleaned from the data are reliable and actionable. This section delves into the importance of data integrity in analytics audits, highlighting potential issues, assessment techniques, and methods for maintaining data quality throughout the process.Data integrity, in the context of analytics audits, is more than just the absence of errors.
It encompasses the accuracy, completeness, consistency, and timeliness of the data used in the audit. This meticulous attention to detail is crucial to produce reliable results. Compromised data integrity can lead to skewed interpretations and unreliable conclusions, potentially impacting business decisions and strategies.
Critical Role of Data Integrity
Data integrity is paramount in analytics audits because it underpins the validity of all subsequent analyses. Inaccurate or incomplete data can lead to misleading conclusions, resulting in poor decision-making. An audit relying on faulty data will likely produce flawed recommendations and fail to identify actual issues or opportunities. Maintaining data integrity directly translates into a more trustworthy and actionable audit report.
Data Integrity Issues
Several factors can compromise data integrity during an audit. These issues can stem from various sources, including data entry errors, inconsistencies in data formats, or missing values. Inaccurate data entry, for instance, can result in incorrect calculations and skewed visualizations, leading to flawed insights. Data inconsistencies between different systems or datasets can create discrepancies that can invalidate the audit’s findings.
Inconsistent data formats, like varying date formats or different units of measurement, also pose a significant challenge.
Assessment and Validation Techniques
Validating data integrity involves a multi-faceted approach. Techniques used include data profiling, which involves analyzing the characteristics of the data, identifying potential anomalies, and detecting inconsistencies. Data cleansing procedures are vital for removing errors and inconsistencies. Data validation rules help ensure that data conforms to predefined criteria. These rules can check for things like the correct data types, ranges, and relationships between different data elements.
Finally, data consistency checks help confirm that data adheres to established business rules. These techniques collectively ensure the reliability of the data used in the audit.
Ensuring Data Quality During the Audit Process
Maintaining data quality throughout the audit process requires proactive measures. This includes establishing clear data governance policies, training staff on data entry procedures, and implementing automated checks to validate data integrity at each stage of the audit. Regular data quality assessments, like performing data profiling or validation, can help identify and correct errors before they significantly impact the audit.
Furthermore, establishing clear communication channels and protocols for data exchange between different teams or systems can help maintain data integrity throughout the audit.
Potential Data Integrity Problems and Their Impact
Problem Type | Description | Impact on Audit | Mitigation Strategies |
---|---|---|---|
Inconsistent Data Formats | Different formats for dates, currency, or other data elements. | Misinterpretations, incorrect calculations, and difficulty in comparing data across sources. | Standardize data formats across all systems and datasets. Use data transformation tools to ensure uniformity. |
Missing Values | Absence of crucial data points in the dataset. | Incomplete analysis, inaccurate conclusions, and potential bias in the audit results. | Identify missing data patterns, impute missing values using appropriate statistical methods, or exclude records with missing data from analysis. |
Data Entry Errors | Typos, incorrect values, or mismatched data during entry. | Incorrect calculations, inaccurate reporting, and skewed audit findings. | Implement data validation rules at the source, use automated data entry tools, and provide comprehensive training to data entry personnel. |
Inconsistent Data Definitions | Different meanings assigned to the same data elements in various systems. | Misinterpretations of data, incorrect comparisons, and discrepancies in findings. | Establish standardized data dictionaries and definitions across all relevant systems. |
Auditing Data Collection Processes
Data collection forms the bedrock of any meaningful analysis. A robust and reliable data collection process is crucial for ensuring the integrity of the entire analytical pipeline. Flaws in this initial stage can propagate through the entire process, leading to inaccurate conclusions and potentially costly errors. Understanding the methods used to assess the effectiveness of data collection is paramount to maintaining data quality.Thorough audits of data collection processes are essential to identify potential weaknesses and ensure the data used for analysis is accurate, complete, and consistent.
This involves examining the methodology employed, identifying potential errors, and implementing strategies to enhance data quality.
Maintaining data integrity in analytics audits is crucial, especially when examining significant shifts in market trends. For instance, understanding how Amazon’s sales and product listings changed during the pandemic, as detailed in Amazon trends during COVID , requires meticulous analysis. This kind of in-depth investigation highlights the importance of consistent and accurate data for reliable conclusions during periods of significant market upheaval, directly impacting the validity of any analytics audit.
Methods for Assessing Data Collection Effectiveness
Data collection effectiveness is evaluated by a variety of methods, each designed to pinpoint specific aspects of the process. These methods include:
- Reviewing Documentation: Examining the data collection protocols, forms, and procedures to assess their completeness, clarity, and adherence to established standards. This includes checking for defined data validation rules and procedures for handling missing data.
- Observing Data Collection Activities: Directly observing the process in action allows for a real-time assessment of how the data is being collected. This observation can reveal discrepancies between documented procedures and actual practice, providing valuable insights into the implementation gap.
- Sampling Data: Randomly selecting a subset of collected data to analyze its quality, completeness, and accuracy. This allows for efficient assessment without the need to scrutinize every data point.
- Interviewing Data Collectors: Gathering feedback from individuals involved in data collection helps identify potential pain points, bottlenecks, and challenges they face in applying the established protocols.
Common Data Collection Errors and Their Implications
Errors in data collection can significantly impact the reliability of analytical findings. Common errors include:
- Inaccurate Data Entry: Typos, incorrect values, or inconsistencies in the data can lead to skewed results and flawed interpretations.
- Incomplete Data: Missing data points can make it difficult to draw complete and accurate conclusions. The implications depend on the proportion of missing data and the importance of the missing information.
- Data Inconsistency: Variations in data formats, units of measurement, or terminology can complicate analysis and introduce bias.
- Data Bias: Systematic errors that favor certain outcomes or groups can skew the results and lead to inaccurate or misleading interpretations.
- Sampling Bias: A sampling methodology that does not accurately represent the target population can lead to unreliable conclusions about the larger population.
Strategies for Improving Data Collection Practices
Improving data collection practices requires a proactive approach:
- Implementing Robust Training Programs: Equipping data collectors with the necessary skills and knowledge to accurately and consistently collect data according to established protocols. This includes training on data validation rules and handling missing data.
- Developing Clear and Concise Data Collection Instruments: Ensuring that data collection forms are user-friendly, unambiguous, and minimize the potential for errors. This includes clear definitions for all fields and data validation rules.
- Establishing Quality Control Mechanisms: Implementing checks and balances at each stage of the data collection process to ensure data accuracy, consistency, and completeness. Examples include data entry validation rules, cross-referencing, and regular audits.
- Utilizing Data Validation Tools: Employing software tools to automate data validation and error detection during the data entry process.
Evaluating Data Source Integrity
A structured process for evaluating the integrity of data sources is essential for ensuring high-quality data:
- Data Source Identification: Explicitly identifying all data sources used for analysis. This includes both internal and external data sources.
- Data Source Documentation: Comprehensive documentation of data sources, including their origin, format, and any known limitations.
- Data Quality Assessment: Regular assessment of the quality of data from each source, including checking for completeness, accuracy, and consistency.
- Data Source Validation: Verifying the accuracy and reliability of data sources through cross-referencing, comparisons, and independent verification.
Data Collection Methods and Potential Weaknesses
Method | Description | Potential Weaknesses | Mitigation Strategies |
---|---|---|---|
Surveys | Gathering data through questionnaires | Low response rates, self-reported bias, poorly designed questions | Incentivize participation, use clear and concise questions, pilot test survey design |
Interviews | Collecting data through structured or semi-structured conversations | Interviewer bias, respondent fatigue, potential for misinterpretation | Train interviewers to maintain neutrality, limit interview duration, record responses accurately |
Observations | Recording data based on direct observation | Observer bias, potential for missing data, difficulty with large-scale observations | Use multiple observers, develop detailed observation protocols, use appropriate tools |
Data Extraction | Collecting data from existing databases | Data format inconsistencies, missing data, data security concerns | Establish data mappings, implement data validation rules, ensure data access protocols |
Auditing Data Storage and Management
Proper data storage and management is crucial for accurate analytics audits. Without robust procedures in place, data integrity can be compromised, leading to skewed results and flawed conclusions. This section delves into the significance of these practices, highlighting the importance of security, access controls, and data governance in maintaining trustworthy audit trails.Data storage and management practices directly impact the reliability of audit findings.
Effective systems ensure data is readily accessible, secure, and compliant with regulations, allowing auditors to efficiently and thoroughly evaluate the data’s integrity and accuracy. This meticulous approach safeguards against errors, omissions, and manipulation, ultimately leading to more precise and trustworthy audit reports.
Significance of Proper Data Storage and Management
Sound data storage and management practices are fundamental for conducting thorough and reliable audits. These practices ensure data integrity, accessibility, and security, all of which are essential components of a successful audit. Poorly managed data can lead to errors, inconsistencies, and difficulties in retrieving the necessary information, which can hinder the audit process and compromise its objectivity.
Importance of Data Security and Access Controls
Robust data security and access controls are paramount during audits. Unauthorized access or modification of data can compromise the accuracy and reliability of audit results. Implementing strict access controls, encryption, and data masking techniques is essential for safeguarding sensitive information and maintaining data integrity. Regular security audits and vulnerability assessments are also critical to identify and mitigate potential risks.
Examples of Data Storage and Management Issues
Several issues related to data storage and management can significantly impact audit results. For instance, outdated or incompatible systems may render data inaccessible or difficult to analyze. Insufficient data backups or inadequate disaster recovery plans can lead to data loss, which would compromise the audit’s scope and effectiveness. Lack of proper version control mechanisms can introduce inconsistencies and make it challenging to track changes to the data.
Improper data classification and tagging can lead to misidentification and incorrect data use, ultimately jeopardizing the entire audit process.
Ensuring accurate data is crucial for any business, especially when it comes to analytics audits and data integrity. With the recent news that selling on Google Shopping is now free, now free to sell on google shopping platform , businesses need to carefully evaluate how this new platform will impact their current data collection and reporting strategies.
Robust analytics audits and data integrity checks are more important than ever to ensure accurate insights and avoid costly mistakes in this new landscape.
Role of Data Governance in Maintaining Data Integrity
Data governance plays a critical role in maintaining data integrity throughout the audit process. A well-defined data governance framework establishes clear policies and procedures for data management, access, and security. This framework ensures data quality, consistency, and compliance with relevant regulations. Data governance also helps maintain audit trails, enabling auditors to track data modifications and verify the integrity of the audit process.
This accountability is essential for building trust in the audit results.
Comparison of Data Storage Solutions
Solution Type | Features | Security Measures | Cost |
---|---|---|---|
Relational Databases (e.g., MySQL, PostgreSQL) | Structured data storage, robust querying capabilities, ACID properties (Atomicity, Consistency, Isolation, Durability) | Access controls, encryption, auditing logs | Moderate to high, often dependent on licensing and maintenance |
NoSQL Databases (e.g., MongoDB, Cassandra) | Flexible schema, scalability, handling of large datasets, fast data retrieval | Authentication, authorization, encryption, access control lists | Variable, often lower than relational databases for specific use cases |
Cloud Storage (e.g., AWS S3, Azure Blob Storage) | Scalability, accessibility, redundancy, cost-effectiveness (often pay-as-you-go) | Encryption at rest and in transit, access controls, multi-factor authentication | Generally low to moderate, based on usage and storage requirements |
Data Warehouses (e.g., Snowflake, Google BigQuery) | Centralized data storage, optimized for analytical queries, data transformation capabilities | Data masking, access controls, audit logs | High, often requiring specialized expertise and ongoing maintenance |
Data storage solutions vary in their features, security measures, and associated costs. Choosing the right solution depends on specific audit needs, data volume, and budget constraints. It’s crucial to evaluate each option thoroughly to ensure it aligns with the audit’s requirements for security, scalability, and cost-effectiveness.
Auditing Data Transformation and Analysis Processes
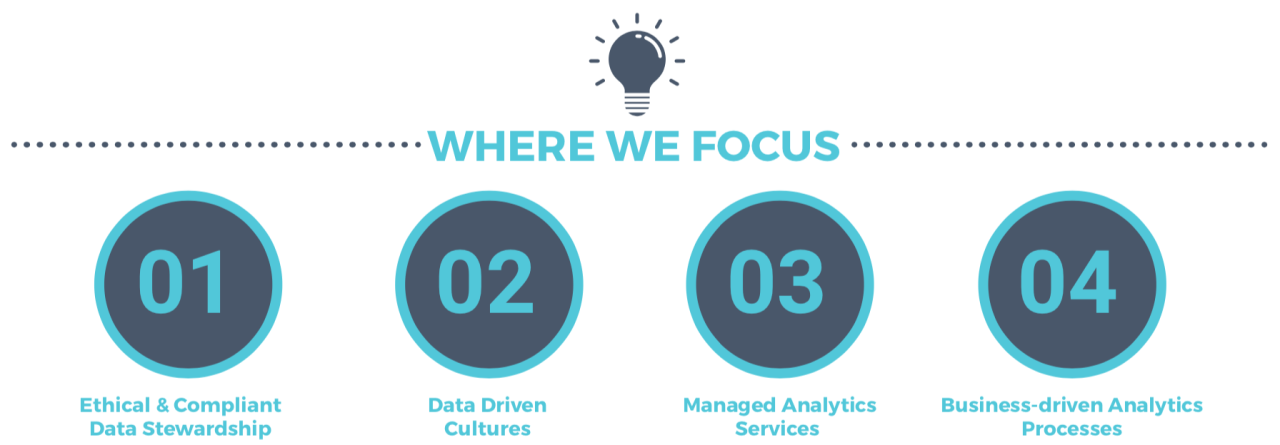
Data transformation and analysis are critical steps in deriving meaningful insights from raw data. Robust auditing of these processes is essential to ensure accuracy, reliability, and compliance. Without proper scrutiny, errors can propagate through the entire analytics pipeline, leading to flawed conclusions and potentially costly decisions. This meticulous review process safeguards against incorrect interpretations and guarantees the integrity of the analytical outputs.
Importance of Reviewing Data Transformation and Analysis Procedures
Data transformation and analysis procedures are not simply a technical exercise; they are integral to the entire analytical process. Thorough review ensures that these processes are correctly implemented, minimizing errors and maximizing the validity of the results. Identifying and addressing potential issues early on prevents the downstream consequences of inaccurate data. Furthermore, adhering to documented procedures promotes consistency and reproducibility, making the analysis more transparent and trustworthy.
Common Errors in Data Transformation and Analysis
Several errors can undermine the reliability of data transformation and analysis results. Incorrect data type mappings, inappropriate aggregation methods, and flawed logic in calculations are common pitfalls. Missing or inconsistent data handling, incorrect data cleansing rules, and improper use of statistical techniques can also introduce significant biases. These issues can lead to skewed insights and ultimately, wrong conclusions.
Carefully reviewing the transformation and analysis steps is crucial to catch these errors.
Strategies for Validating Data Transformations and Analytical Outputs
Validation strategies are vital for verifying the accuracy and reliability of the transformed data and analytical outputs. Comparing transformed data with original data using statistical tests is essential. Reviewing data distributions and summaries before and after transformation helps to identify potential issues. Furthermore, applying different analytical techniques to the same data and comparing the results can help in identifying inconsistencies and confirming the validity of the findings.
Structured Method for Reviewing Data Analysis Workflows
A structured method for reviewing data analysis workflows is critical for maintaining consistency and minimizing errors. This method should involve a checklist of key steps in the data transformation and analysis pipeline. The review should include verification of the input data quality, scrutiny of the transformation steps, validation of intermediate results, and evaluation of the final analytical outputs.
Ensuring data integrity in analytics audits is crucial. A key part of that process is making sure your data is easily understood by search engines, and using structured data markup like use question answer schema markup can significantly improve how search engines interpret your data. This, in turn, leads to better accuracy and efficiency in your analytics audits, and ultimately, more insightful results.
This structured approach will ensure that all aspects of the process are thoroughly examined.
Table of Common Data Transformation Steps and Potential Issues
Step | Description | Potential Errors | Mitigation Techniques |
---|---|---|---|
Data Loading | Transferring data from source systems to the target system. | Incorrect data format, missing data, inconsistencies in data types. | Data validation rules, data profiling tools, data quality checks. |
Data Cleaning | Handling missing values, outliers, and inconsistencies in the data. | Inappropriate imputation methods, incorrect outlier removal strategies, data leakage. | Statistical methods for imputation, robust outlier detection, data masking techniques. |
Data Transformation | Converting data into a usable format for analysis. | Incorrect data type conversion, loss of information during transformation, unintended aggregation. | Data mapping specifications, data validation rules, comprehensive documentation of transformations. |
Data Aggregation | Combining data from multiple sources or levels. | Incorrect aggregation logic, overlooking crucial variables, misinterpretation of aggregated data. | Clear aggregation rules, careful selection of variables, verification of aggregation results. |
Data Analysis | Applying analytical techniques to the transformed data. | Inappropriate statistical methods, selection bias, incorrect interpretation of results. | Statistical knowledge, domain expertise, rigorous validation of results, replication of analysis. |
Tools and Technologies for Analytics Audits
Analytics audits are crucial for ensuring data quality and reliability. A key component of these audits is the utilization of appropriate tools and technologies. These tools automate tasks, streamline processes, and offer insights that would be extremely time-consuming or impossible to achieve manually. Effective analytics audit tools are essential for maintaining data integrity, identifying anomalies, and ultimately, generating trustworthy insights from the data.
Software Tools for Analytics Audits
A wide range of software tools are available for performing analytics audits. These tools vary in their capabilities and functionalities, but all aim to improve the efficiency and effectiveness of the audit process. They enable auditors to systematically assess various aspects of the analytics pipeline, including data collection, storage, transformation, and analysis. The selection of appropriate tools depends on the specific needs and scope of the audit.
Commonly Used Tools
A range of software tools are commonly employed in analytics audits. These tools assist in various aspects of the audit process, including data profiling, validation, and anomaly detection. They help to ensure data quality and consistency across the entire analytics lifecycle. Popular choices include open-source and commercial options, catering to diverse needs and budgets.
- Data Profiling Tools: Tools like Alation, Dataiku, and Informatica PowerCenter provide capabilities for understanding data characteristics, including data types, formats, and quality. They assist in identifying potential issues and inconsistencies early in the audit process.
- Data Validation Tools: These tools are designed to check data against predefined rules and constraints. Examples include Apache Spark and Talend. They help ensure data conforms to business rules and expectations, minimizing errors and inconsistencies.
- Data Quality Management Platforms: Platforms like Qlik Sense, Tableau, and Power BI allow auditors to monitor data quality over time. They enable tracking metrics like data accuracy, completeness, and consistency. Auditors can also identify trends and patterns in data quality issues.
- SQL Querying and Reporting Tools: Tools like SQL Developer, Toad, and MySQL Workbench are used to interrogate databases and generate reports. These tools are essential for data validation and verification in analytics audits.
Features and Functionalities
These tools offer a variety of features to support analytics audits. These features typically include:
- Data Profiling: Identifying data characteristics like missing values, outliers, and inconsistencies. Tools often provide visualizations and reports for easy interpretation.
- Data Validation: Ensuring data meets predefined criteria and business rules. Automated checks for data accuracy, completeness, and consistency are common.
- Data Lineage Tracking: Mapping data sources, transformations, and destinations. This helps understand the flow of data through the analytics pipeline.
- Anomaly Detection: Identifying unusual patterns or deviations in data. This can help detect errors, fraud, or other issues.
- Reporting and Visualization: Presenting audit findings in a clear and understandable format. Tools often offer dashboards and reports to summarize key insights.
Detailed Description of a Data Quality Tool
Data quality management platforms, like Informatica Data Quality, are specifically designed to assess and improve data quality. They provide a comprehensive suite of tools for data profiling, validation, and cleansing. Key functionalities include:
- Automated data profiling: Quickly assess the quality of data by identifying characteristics, such as data types, formats, and ranges.
- Data validation rules: Implement predefined rules and constraints to ensure data accuracy and consistency.
- Data cleansing and transformation: Address data quality issues by correcting errors, handling missing values, and standardizing data formats.
- Data quality dashboards: Visualize key data quality metrics and trends, enabling proactive monitoring and issue resolution.
Comparison of Analytics Audit Tools
Tool Name | Key Features | Data Types Supported | Pricing |
---|---|---|---|
Informatica Data Quality | Automated profiling, validation, cleansing, dashboards | Various structured and unstructured data | Typically licensed based on users and features |
Alation | Data discovery, lineage tracking, data governance | Structured and semi-structured data | Typically subscription-based |
Dataiku | Data preparation, modeling, machine learning | Structured and semi-structured data | Subscription-based, tiered pricing |
Talend | Data integration, transformation, quality management | Various data formats | Subscription-based, tiered pricing |
Reporting and Documentation in Audits
Comprehensive reporting is crucial in analytics audits. It’s not just about documenting the findings; it’s about communicating them effectively to stakeholders, highlighting areas needing improvement, and demonstrating the value of the audit itself. Clear and concise reporting facilitates action and ensures that audit recommendations are not simply filed away.Effective reporting in analytics audits involves a structured approach to documenting the audit process, findings, and recommendations.
This process ensures that all critical aspects of the audit are captured and communicated effectively, facilitating the implementation of necessary improvements and preventing future issues. Clear documentation of audit procedures and findings is paramount for maintaining transparency, accountability, and the ability to replicate the audit in the future.
Importance of Comprehensive Reporting
Effective reporting in analytics audits ensures that the audit process’s value is recognized by stakeholders and that recommendations are actionable. Detailed reporting enhances transparency and accountability, enabling the organization to understand the areas needing improvement. This transparency builds trust and allows for better decision-making regarding data management and analysis processes.
Structure and Content of Audit Reports
Audit reports should follow a structured format to ensure clarity and completeness. Typically, an audit report includes an executive summary, a detailed description of the audit methodology, a comprehensive list of findings, and specific recommendations. The executive summary provides a concise overview of the audit’s scope, key findings, and recommendations. The methodology section details the steps taken during the audit, such as data collection, analysis, and testing.
Findings are presented in a clear and concise manner, including relevant data and supporting evidence. Recommendations should be actionable and provide clear steps for improvement. Specific examples of recommendations include data validation procedures, data quality improvement strategies, or changes to data transformation pipelines.
Importance of Clear Documentation of Audit Procedures and Findings
Clear documentation of audit procedures and findings is essential for several reasons. Firstly, it provides a complete record of the audit process, allowing for future reference and replication. Secondly, it helps to ensure consistency in audit practices across different audits. Thirdly, it provides a basis for demonstrating the audit’s effectiveness and the implementation of corrective actions. Well-documented procedures enhance accountability and provide a historical record of data integrity.
Example of an Audit Report
Executive Summary: This audit assessed the data integrity of the customer relationship management (CRM) system. The audit revealed several inconsistencies in data entry and a lack of validation procedures. Actionable recommendations for data cleansing and improved validation procedures are Artikeld.
Audit Methodology: The audit involved data sampling from the CRM database, review of data entry forms, and interviews with data entry personnel. Data validation rules and procedures were examined.
Findings:
- Inconsistent data entry was observed for customer addresses, leading to potential errors in customer segmentation.
- Lack of validation procedures for email addresses resulted in duplicate entries and invalid email addresses.
- Incomplete data fields for customer demographics were found in 15% of the sampled data.
Recommendations:
- Implement data validation rules for customer addresses, email addresses, and demographics to prevent data entry errors.
- Develop a standardized data entry training program for data entry personnel.
- Schedule a monthly data quality review to monitor the effectiveness of the implemented validation rules and procedures.
Key Components of an Analytics Audit Report
Section | Description | Purpose | Example |
---|---|---|---|
Executive Summary | Concise overview of the audit, key findings, and recommendations. | Provides a high-level understanding of the audit for stakeholders. | “This audit assessed the accuracy of sales data. Key findings included inconsistencies in product pricing and a lack of data validation rules.” |
Audit Methodology | Detailed description of the audit procedures and techniques. | Provides transparency and allows for replication. | “Data sampling from the sales database, review of sales order forms, and interviews with sales representatives.” |
Findings | Clear and concise presentation of inconsistencies and issues identified. | Highlights areas needing improvement. | “Discrepancies in product pricing were found in 10% of the sampled sales data.” |
Recommendations | Actionable steps to address the identified issues. | Provides clear guidance for improvement. | “Implement a new pricing validation rule in the sales order system.” |
Outcome Summary
In conclusion, meticulous analytics audits and robust data integrity practices are vital for any organization striving for data-driven success. By understanding the different facets of these audits, from defining the scope to using appropriate tools and technologies, you can build a strong foundation for reliable and actionable insights. This comprehensive guide provides a practical framework for implementing and maintaining these practices, enabling you to leverage data effectively and make informed decisions.