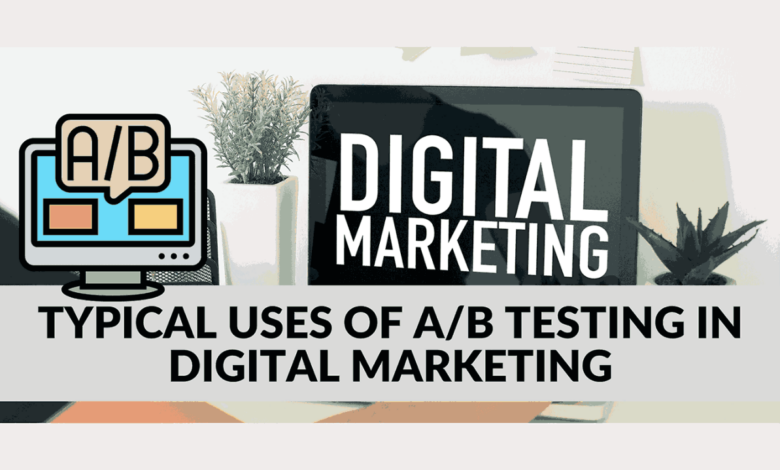
A/B Testing Marketing Explained Maximize Your Campaigns
A b testing marketing explained – A/B testing marketing explained is crucial for optimizing your marketing campaigns. It’s a scientific approach to refining your strategies, ensuring your efforts are yielding the best results. This guide delves into the fundamentals, from selecting the right variables to interpreting data and implementing changes. Learn how to use data-driven insights to boost conversions, engagement, and ultimately, your bottom line.
We’ll cover everything from defining A/B testing and its core principles to setting up and implementing your own tests, analyzing the results, and optimizing your campaigns for continuous improvement. This comprehensive guide will equip you with the knowledge and tools to make informed decisions and see real results from your marketing initiatives.
Introduction to A/B Testing
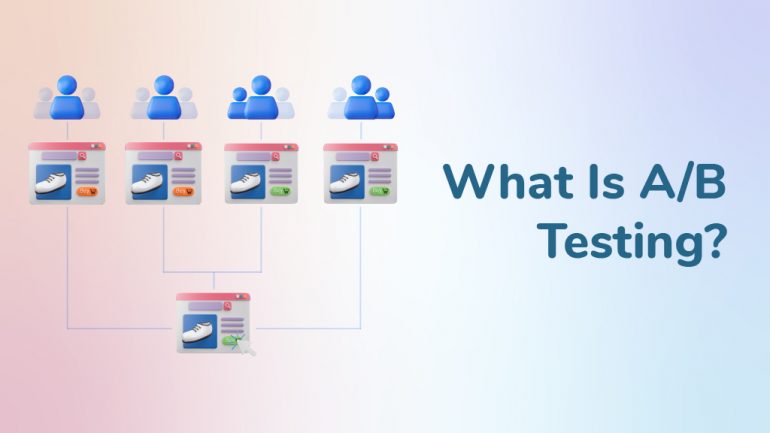
A/B testing is a cornerstone of modern marketing. It’s a systematic approach to comparing two versions of a marketing element, such as a webpage, advertisement, or email, to determine which performs better. This iterative process allows marketers to optimize their campaigns and maximize their return on investment (ROI). By meticulously testing different variations, businesses can gain insights into what resonates best with their target audience, ultimately leading to improved conversions and engagement.A/B testing relies on the fundamental principle of rigorous experimentation.
It involves carefully defining a specific hypothesis, developing variations, and then analyzing the results to determine the optimal version. This methodical approach is crucial for making data-driven decisions, moving away from guesswork and intuition. The key is to isolate the variable being tested and measure its impact on the desired outcome, whether that’s clicks, conversions, or brand awareness.
Key Objectives of A/B Testing
A/B testing in marketing campaigns aims to achieve several critical objectives. These include increasing conversion rates, boosting engagement metrics, improving user experience, and ultimately driving revenue growth. By identifying the most effective elements, businesses can streamline their marketing efforts and generate more profitable outcomes. This data-driven optimization allows for continuous improvement and adaptation to evolving consumer preferences.
Types of A/B Tests, A b testing marketing explained
Understanding the different types of A/B tests is crucial for effective campaign optimization. These tests are designed to isolate specific elements and measure their impact on key metrics. By meticulously analyzing the results of various tests, businesses can gain valuable insights into what works best for their target audience.
Test Type | Example | Variation | Objective |
---|---|---|---|
Button Color | Call to Action Button | Red button vs. Green button | Determine which button color generates more clicks and conversions. |
Headline Variations | Ad copy | Short, punchy headline vs. Descriptive headline | Identify which headline better captures attention and drives interest. |
Landing Page Design | Website landing page | Clean, minimalist design vs. Visually rich design | Measure the impact of different design elements on conversion rates. |
Email Subject Lines | Email campaign | Intriguing subject line vs. Straightforward subject line | Optimize email open rates and click-through rates. |
Setting Up A/B Tests
A/B testing is a powerful tool for optimizing marketing campaigns, but its effectiveness hinges on meticulous setup. This phase involves careful selection of variables, appropriate metrics, and a statistically sound sample size. A well-structured framework, coupled with the right tools, maximizes the potential of your tests.
Selecting Variables for A/B Testing
The key to successful A/B testing lies in identifying the right variables to modify. These variables, often related to elements of your marketing materials, should directly impact the desired outcome. Consider aspects like headlines, call-to-actions, images, colors, and even the placement of elements on a webpage. Focusing on a limited set of variables allows for a clearer understanding of which changes produce the desired effect.
For example, testing the headline alone will give you more precise data about its influence than testing headlines, call-to-actions, and images simultaneously.
Choosing Appropriate Metrics for Measurement
Selecting the right metrics is crucial for evaluating the success of your A/B tests. These metrics should directly reflect the goals of your campaign. Common metrics include conversion rates, click-through rates, bounce rates, average order value, and customer lifetime value. Conversion rate, for instance, is a vital metric for e-commerce businesses, reflecting the percentage of visitors who complete a purchase.
Choose metrics that align with your specific business objectives.
Defining a Statistically Significant Sample Size
Determining the appropriate sample size is critical to ensuring the reliability of your A/B test results. A sample size that is too small may yield unreliable results, while one that is excessively large can be wasteful. Statistical power analysis tools and calculators can assist in determining the necessary sample size needed to achieve a statistically significant result. This calculation considers factors such as the expected effect size, desired confidence level, and the acceptable margin of error.
For instance, if you’re testing a new landing page, a sample size of 100 visitors might not be enough to detect a significant difference, whereas 500 or more might provide more robust and reliable results.
Setting Up an A/B Testing Framework
A well-defined A/B testing framework ensures that the testing process is systematic and reproducible. This involves clearly outlining the testing goals, defining the variables, selecting the appropriate metrics, and determining the sample size. The framework should also specify the duration of the test and the criteria for declaring a winner. A detailed protocol ensures consistent execution and data interpretation.
Examples of Tools and Platforms Used for A/B Testing
Numerous tools and platforms facilitate A/B testing. Google Optimize, Optimizely, and VWO are popular choices, offering comprehensive features for creating and managing A/B tests. These tools typically allow for easy setup, visualization of results, and integration with other marketing platforms. Each tool may have different pricing models, support levels, and feature sets. For example, Google Optimize is a free option for smaller businesses, while Optimizely offers more advanced features for larger organizations.
Choosing the right tool depends on your specific needs and budget.
Implementing A/B Tests
A/B testing isn’t just about choosing between two options; it’s about iteratively refining your marketing strategies. Once you’ve defined your hypotheses and set up your tests, the next crucial step is implementing them effectively. This involves creating variations of your original content, strategically allocating traffic, and meticulously monitoring the results.Implementing a successful A/B test requires a methodical approach to ensure accurate results and actionable insights.
This process involves careful planning and execution, from crafting compelling variations to tracking key metrics. Thorough implementation is essential for drawing meaningful conclusions and improving campaign performance.
A/B testing in marketing is all about figuring out what works best, and that often involves trying different approaches. To make the most of your Instagram presence, you should check out these 24 must-have Instagram apps for better posts, 24 must have instagram apps for better posts. Ultimately, understanding your audience and what resonates with them is key to successful A/B testing campaigns.
Creating Variations of Original Content
Effective A/B testing hinges on creating compelling variations that clearly differentiate from the original. Simply swapping a few words isn’t enough; the goal is to identify specific elements that influence user behavior. This could be headlines, call-to-action buttons, image choices, or even the layout of a webpage.A critical component of creating variations is to understand the nuances of user behavior.
Analyzing user journeys and preferences helps tailor variations that resonate more deeply. This could involve testing different colors, fonts, or even the placement of key information on a page. The more targeted and focused the variations, the more accurate and useful the insights derived from the test will be. For instance, a variation could highlight a specific benefit or feature of a product, while another variation focuses on the price or ease of purchase.
Traffic Allocation Strategies
Ensuring that traffic is distributed fairly across the variations is crucial for accurate results. The ideal approach is to use a random allocation method to prevent bias. Tools like A/B testing platforms automatically handle this process, dividing traffic proportionally across variations. This randomness ensures that each variation receives a representative sample of users.This ensures that the results are not skewed by a disproportionate allocation of traffic to one particular variation.
A simple example would be splitting 50% of the traffic to the original and 50% to the variation. Tools can help manage this automatically. Using consistent allocation strategies, you can avoid misinterpreting results due to an uneven distribution of traffic.
A/B testing in marketing is all about figuring out what works best, and understanding your audience is key. To really maximize your online presence and get the most out of your marketing efforts, partnering with expert social media management companies like expert smm companies for online retailers can be a game-changer. Testing different headlines, images, or calls to action, helps you fine-tune your strategy for optimal results, and ultimately drive more sales.
Monitoring and Analyzing Results in Real-Time
Real-time monitoring allows for quick adjustments and course correction during the test. Analyzing key metrics like click-through rates, conversion rates, and bounce rates helps identify trends and make informed decisions. Tools often provide dashboards and reports that visualize these metrics, enabling rapid analysis. Using these tools effectively helps in tracking the progress of the test and making changes as needed.For example, if a variation shows a significant drop in conversion rates, the test can be halted or modified.
Tools that display results in real-time are invaluable in adjusting the test as needed, making it a dynamic process that can quickly adapt to new insights. This enables a more efficient use of resources and time.
Strategies for Handling User Interactions During A/B Testing
The following table Artikels different strategies for handling user interactions during A/B testing.
Strategy | Method | Data Collected | Analysis |
---|---|---|---|
Heatmaps | Visual representation of user activity on a webpage, highlighting areas of interest and clicks. | Click locations, scroll depth, time spent on specific elements. | Identify elements that draw user attention and those that are ignored. |
Click-Through Rates (CTR) | Percentage of users who click on a specific element. | Number of clicks divided by total impressions. | Measure the effectiveness of calls-to-action and other interactive elements. |
Bounce Rates | Percentage of users who leave a webpage after viewing only one page. | Number of single-page visits divided by total visits. | Identify usability issues or elements that discourage users from continuing their journey. |
Conversion Rates | Percentage of users who complete a desired action (e.g., making a purchase). | Number of conversions divided by total visits. | Evaluate the effectiveness of the variation in driving conversions. |
Analyzing A/B Test Results
Interpreting A/B test data is crucial for understanding which variation performs better and making data-driven decisions. A thorough analysis goes beyond simply observing which variation generated more clicks; it requires a deep dive into statistical significance and the potential impact on business goals. This process helps determine if observed differences are genuine or due to random chance.Analyzing A/B test results involves a systematic approach to identify significant differences between variations, accounting for potential biases and limitations in the data.
This process is essential for informed decision-making, preventing costly mistakes, and maximizing the return on investment from marketing campaigns.
Methods for Interpreting A/B Test Data
A key aspect of interpreting A/B test data is understanding statistical significance. Statistical tests determine the probability that observed differences between variations are not due to random chance but are truly representative of a difference in effectiveness. This involves comparing the performance metrics of the variations and calculating a p-value. A low p-value indicates a statistically significant difference.
Identifying Significant Differences
To identify statistically significant differences between variations, various statistical tests are applied. The appropriate test depends on the type of data being analyzed (e.g., numerical or categorical). A thorough understanding of the data’s characteristics is crucial for selecting the correct test.
Avoiding Pitfalls in Interpreting Results
Common pitfalls in interpreting A/B test results include: misinterpreting statistical significance, failing to account for confounding variables, and drawing conclusions from insufficient data. Understanding these potential issues helps ensure the analysis is rigorous and reliable. The focus should be on drawing accurate conclusions from the collected data, and not relying on intuition or subjective opinions.
Comparison of Statistical Tests
Different statistical tests are suitable for different types of data and research questions. A proper understanding of these tests is essential for selecting the appropriate tool for a given situation.
Test | Data Type | Purpose | Example Use Case |
---|---|---|---|
t-test | Numerical data (e.g., conversion rates, average order value) | Compares the means of two groups | Comparing the average conversion rate of two different website designs. |
Chi-squared test | Categorical data (e.g., clicks, purchases) | Assesses the association between two categorical variables | Determining if there’s a relationship between the color of a button and click-through rates. |
ANOVA (Analysis of Variance) | Numerical data (e.g., average order value, customer satisfaction scores) | Compares the means of three or more groups | Evaluating the effectiveness of three different marketing campaigns on customer satisfaction. |
Regression analysis | Numerical data | Models the relationship between variables | Analyzing the impact of different ad copy variations on conversion rates, considering other factors like demographics or time of day. |
Optimizing Marketing Campaigns: A B Testing Marketing Explained
A/B testing provides valuable insights, but the true power lies in effectively implementing those findings to optimize your marketing campaigns. This involves more than just switching out a button color; it requires a strategic approach to ensure maximum impact across your entire marketing platform. Successfully implementing these changes can lead to a significant improvement in campaign performance and return on investment (ROI).Successfully leveraging A/B testing results necessitates a systematic approach that goes beyond simply identifying winning variations.
It involves understanding how these variations will integrate into existing marketing workflows and how to effectively scale the improvements. This approach prioritizes a seamless transition and avoids disrupting existing strategies.
Implementing Winning Variations
Implementing the winning variations from A/B tests requires a meticulous approach to ensure a smooth transition and minimize disruption. This involves careful planning and execution across all relevant channels.
- Strategic Rollout: Plan a phased rollout of the winning variations, starting with a small, controlled group of users or customers. This allows for monitoring and adjustments before fully implementing the change across the entire platform. This “pilot” phase allows for identification of potential issues or unforeseen consequences in a contained environment.
- Cross-Platform Integration: Ensure seamless integration across all your marketing channels. If the winning variation involves a change to your website, for example, ensure that the change is also reflected in your social media posts, email marketing campaigns, and other relevant platforms. This consistent messaging is crucial for maintaining a unified brand experience.
- Training and Communication: Provide training to your marketing team on the implemented changes. Clear communication is essential to ensure everyone understands the rationale behind the changes and how to effectively use the new elements. This fosters consistency and avoids confusion among the team.
Ongoing A/B Testing and Iteration
A/B testing isn’t a one-time event; it’s an ongoing process. Continuous testing allows for adjustments based on real-time data and changing market conditions.
- Continuous Monitoring: Track key performance indicators (KPIs) after implementing the winning variations to measure their effectiveness. This will provide insights into the performance of the new elements and identify areas for further optimization. This ongoing monitoring is crucial for understanding the impact and identifying any unforeseen issues.
- Further Testing: Don’t stop testing! Identify new areas for improvement based on the results of the initial A/B tests. This could involve testing different calls to action, headlines, or images to continue optimizing campaign performance. This cycle of testing and improvement allows for constant adaptation to maintain peak performance.
- Adapting to Market Trends: The market is constantly evolving, and customer preferences change. Ongoing A/B testing enables you to adapt to these trends and maintain a competitive edge. This adaptation ensures that campaigns remain relevant and effective in a constantly shifting landscape.
Best Practices for Continuous Improvement
Implementing a robust A/B testing strategy requires adherence to best practices to maximize its effectiveness.
- Establish Clear Goals: Define specific, measurable, achievable, relevant, and time-bound (SMART) goals for each A/B test. This focus will help in tracking the success and guide the iteration process. This is the foundation for measuring success.
- Data-Driven Decisions: Base your decisions on data and analytics rather than assumptions or intuition. This approach ensures that changes are based on concrete evidence and not on subjective opinions.
- Test Frequently: Don’t wait for significant changes in performance to run tests. Regular A/B testing allows for small, incremental improvements that can accumulate to significant results over time.
Illustrative Examples
A/B testing isn’t just a theoretical concept; it’s a powerful tool used daily by marketers to optimize campaigns. Understanding real-world examples, both successful and unsuccessful, is crucial for effective implementation. These examples illustrate how to identify opportunities for improvement, and how to avoid common pitfalls.Successful A/B testing relies on meticulous planning, precise execution, and a thorough analysis of the results.
A/B testing marketing is all about experimenting with different versions of your campaigns to see what performs best. Knowing how to craft compelling subject lines and personalize email content is crucial for success. Learning effective email marketing strategies, like those found in successful tips and strategies for email marketing , can significantly improve your results. Ultimately, understanding what resonates with your audience through A/B testing is key to maximizing your marketing efforts.
This section will delve into specific case studies to highlight these key elements.
A Successful A/B Test Case Study
A retail company tested two different email subject lines for a promotional offer. The first subject line, “Don’t Miss Out!” used a sense of urgency, while the second, “Exclusive Offer Just for You,” focused on personalization. The results showed a 15% increase in open rates for the “Exclusive Offer” email, and a 20% increase in click-through rates. This success was attributed to the more engaging and personalized nature of the second subject line, which resonated better with the target audience.
The company adjusted its email marketing strategy, leading to improved engagement and conversions.
A Failed A/B Test Example
A software company tested a new, modern landing page design against their existing design. The new design included bold colors and a more abstract layout. While the new design was visually appealing to the company’s designers, the test showed a significant decrease in conversion rates. Analysis revealed that the new design was more difficult to navigate and the call-to-action buttons were less prominent.
The company learned that visual appeal isn’t enough; user experience and usability are critical factors in successful A/B testing. The failure stemmed from a lack of user-centered design in the new design, resulting in a poorer user experience.
A/B Testing for a Website Landing Page
A/B testing a landing page involves systematically changing one element at a time to see how it affects conversions. For example, consider a landing page promoting a new software product. The company could test different headlines, call-to-action buttons, or even the layout of the page.
- Headline Testing: The company could test two different headlines, one emphasizing ease of use and the other highlighting the product’s unique features. A/B testing can reveal which headline resonates more with the target audience and leads to higher conversion rates.
- Call-to-Action (CTA) Testing: A/B testing can be used to determine the optimal CTA. Two different CTAs (e.g., “Get Started Now!” vs. “Learn More”) can be compared to see which drives more conversions.
- Layout and Visual Elements Testing: Testing the layout of the page and visual elements, like images and colors, is also important. Different layouts can be tested to determine which one is more engaging and conducive to conversions.
A/B Testing Email Marketing Campaigns
Testing email marketing campaigns is similar to testing landing pages. Here, the elements to change include subject lines, email content, and call-to-action buttons.
- Subject Line Testing: Test different subject lines to see which one performs best. A/B tests can determine if a more personal subject line or a more straightforward subject line achieves higher open rates.
- Email Content Testing: Test variations in the body of the email, such as the tone, the level of detail, and the use of visuals. This could involve testing a concise email versus a detailed email, or an email with a lot of images vs. a minimal-image email.
- Call-to-Action (CTA) Button Testing: Test variations in the CTA buttons, like color, size, and wording, to see which performs better. An A/B test could compare a button that says “Buy Now” to a button that says “Learn More”.
Common Challenges and Considerations
A/B testing, while powerful, isn’t without its hurdles. Understanding potential obstacles and how to navigate them is crucial for successful campaign optimization. Knowing how to anticipate and overcome these challenges ensures that your efforts yield meaningful results and avoid wasted resources. This section dives into common pitfalls and provides strategies to address them effectively.
Common Obstacles in A/B Testing
A/B testing often encounters roadblocks that can hinder the effectiveness of the process. These challenges, if not addressed, can lead to misleading conclusions and ineffective marketing strategies. Understanding these obstacles is the first step toward overcoming them.
- Insufficient Sample Size: A common mistake is running tests with too few participants. This can lead to unreliable results, as the observed differences might be due to random chance rather than a genuine effect of the tested variable. Increasing the sample size ensures that the results accurately reflect the population and are statistically significant.
- Incorrectly Defined Metrics: Focusing on the wrong metrics can lead to flawed conclusions. For instance, a campaign might see an increase in page views but a decrease in conversions. This highlights the importance of defining clear and relevant metrics that align with the overall business objectives. Precisely measuring conversions, click-through rates, and other relevant KPIs is vital for effective analysis.
- Unrealistic Expectations: Expecting significant improvements in every A/B test is unrealistic. A/B testing is a process of incremental improvement, not a guarantee of radical transformations. Understanding this nuanced aspect is key to maintaining realistic expectations and consistent effort. Real-world results often demonstrate that not every variation will lead to substantial gains.
- Confounding Variables: External factors unrelated to the tested variables can impact the results. For example, seasonal trends or changes in market conditions might affect user behavior. Careful consideration of these external factors is crucial for interpreting results accurately. Using controls to isolate the impact of the specific variables being tested is important.
Strategies for Overcoming Challenges
Effective strategies are essential for mitigating the obstacles inherent in A/B testing. Addressing these obstacles with well-defined plans leads to a more reliable and insightful approach to campaign optimization.
- Employ Statistical Power Analysis: Determining the appropriate sample size before initiating an A/B test is crucial for achieving statistically significant results. This ensures that the test is powerful enough to detect a meaningful difference, if one exists. Statistical power analysis helps establish a sufficient sample size.
- Prioritize User Experience (UX): Changes should focus on improving user experience, not just on superficial aesthetics. Ensuring the usability and intuitive nature of the variations is critical. A good UX design improves user satisfaction, leading to better engagement and conversions.
- Employ a Controlled Testing Environment: Isolate the tested variables to avoid confounding factors. Consider factors like time of day, user demographics, and external events. Strict control over variables allows for a more accurate assessment of the impact of the tested elements.
- Iterative Testing: Avoid expecting dramatic results from a single A/B test. Instead, adopt an iterative approach, testing variations in small increments, and continually refining the best-performing versions. This iterative approach builds on successes and gradually improves campaign performance.
Importance of User Experience (UX) in A/B Testing
User experience (UX) is paramount in A/B testing. Changes should not compromise user satisfaction, which can result in negative feedback or a lack of engagement. A positive user experience can translate into better engagement and conversions.
- Prioritize User Needs: Modifications to the user interface should always align with user needs and expectations. A deep understanding of user behavior helps determine the most effective design solutions. A user-centered design approach leads to improved satisfaction.
- Focus on Intuitive Navigation: Ensure that the design elements are easy to understand and use. Complicated layouts and confusing navigation can lead to a poor user experience. User-friendly navigation promotes user satisfaction and engagement.
- Optimize for Mobile Devices: With the rise of mobile users, it’s critical to ensure that the variations are optimized for mobile devices. A poor mobile experience can significantly reduce user engagement. Optimizing for mobile devices is crucial for broader reach and user engagement.
Selecting the Right A/B Testing Tools
Choosing the right A/B testing tools is crucial for success. The chosen tool should align with the scale and complexity of the tests. Considerations include ease of use, reporting capabilities, and integrations.
- Consider Ease of Use and Functionality: Look for tools with user-friendly interfaces, intuitive dashboards, and a clear reporting structure. The ease of use allows for seamless implementation and analysis. A user-friendly tool ensures efficiency and minimizes learning curves.
- Assess Reporting Capabilities: Tools with detailed reporting and visualization capabilities are essential for analyzing results effectively. This enables a clear understanding of the impact of changes. Comprehensive reports offer valuable insights into performance metrics.
- Check for Integrations: Ensure compatibility with existing marketing platforms and systems. This streamlines the testing process and avoids data silos. Integration ensures seamless data flow and efficient management.
Future Trends in A/B Testing
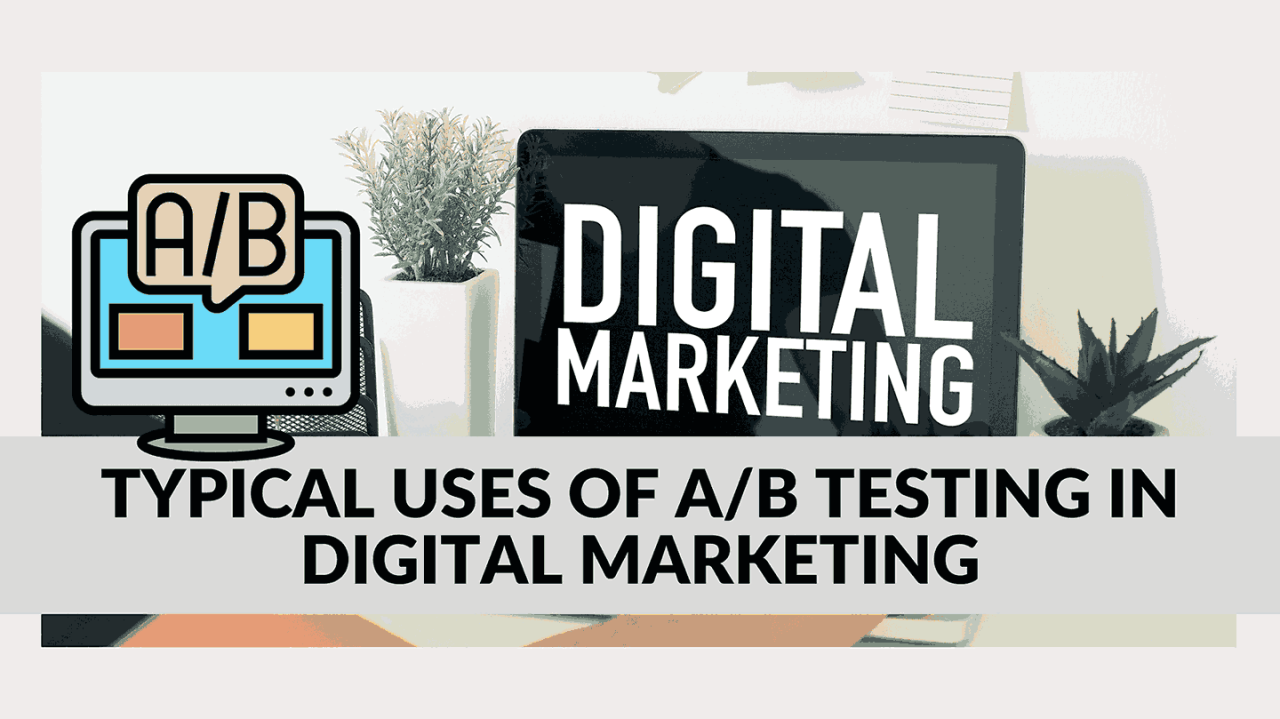
A/B testing, while a mature methodology, is constantly evolving. Emerging technologies and data-driven insights are reshaping how marketers approach campaign optimization. This section explores the exciting future of A/B testing, focusing on AI integration, personalized experiences, and the role of machine learning in enhancing testing strategies.The future of A/B testing hinges on the ability to gather and analyze vast amounts of data more efficiently and to leverage sophisticated algorithms to predict optimal outcomes.
This means moving beyond simple hypothesis testing to more nuanced and predictive models.
AI-Powered A/B Testing
AI is transforming A/B testing by automating complex processes and enabling more sophisticated analysis. AI algorithms can identify patterns and correlations in data that might be missed by traditional methods. This allows for faster identification of winning variations and more efficient optimization cycles.
- Predictive Modeling: AI algorithms can predict the likelihood of a variation’s success based on historical data, user behavior, and other relevant factors. This allows marketers to prioritize testing variations with the highest potential for positive impact.
- Automated Experiment Design: AI can automatically suggest the most effective experiments based on existing data and business goals. This streamlines the testing process, reducing manual effort and improving efficiency.
- Real-time Optimization: AI can analyze user interactions in real-time during an A/B test, enabling dynamic adjustments to the test parameters. This ensures the test is focused on the most relevant user segments and maximizes the likelihood of finding the best performing variation.
Personalization and A/B Testing
Personalization is becoming increasingly important in marketing, and A/B testing plays a crucial role in achieving it. By tailoring content and offers to individual user preferences, businesses can enhance engagement and conversions. This trend will continue to grow as consumers expect more personalized interactions.
- Segment-Specific Testing: A/B testing can be used to identify the most effective messages and offers for different customer segments. This allows for a more targeted and effective approach to personalization.
- Dynamic Content Delivery: AI can be used to dynamically deliver different content variations based on real-time user data. For instance, showing a different product recommendation to a user based on their browsing history. This enables a highly personalized experience for each user.
- Predictive Personalization: Using machine learning models, marketers can predict user needs and preferences to deliver highly relevant content and offers. This proactive approach to personalization anticipates user needs and leads to higher engagement.
Machine Learning in A/B Testing Strategies
Machine learning algorithms can analyze vast amounts of data to identify patterns and relationships that might be missed by traditional A/B testing methods. This enables the development of more sophisticated and accurate predictive models.
- Predictive Modeling: Machine learning models can forecast the potential impact of different variations before they are deployed. This enables marketers to make data-driven decisions about which variations to test and prioritize.
- Multi-Variant Testing Optimization: Machine learning can optimize multi-variant tests by identifying the most effective combinations of elements and suggesting variations that complement each other.
- Automated Reporting and Analysis: Machine learning algorithms can automate the process of analyzing A/B test results and generating actionable insights. This saves time and resources, allowing marketers to focus on implementing the findings.
Final Review
In conclusion, mastering A/B testing marketing explained empowers marketers to refine campaigns, optimize conversions, and ultimately achieve greater success. By understanding the process from setup to analysis and continuous improvement, you can transform your marketing strategies into data-driven engines of growth. The key is to remember that A/B testing is not a one-time fix; it’s an ongoing process of learning, adapting, and iterating to achieve optimal results.